View : 251 Download: 0
Similar Image Retrieval using Autoencoder. I. Automatic Morphology Classification of Galaxies
- Title
- Similar Image Retrieval using Autoencoder. I. Automatic Morphology Classification of Galaxies
- Authors
- Seo E.; Kim S.; Lee Y.; Han S.-I.; Kim H.-S.; Rey S.-C.; Song H.
- Ewha Authors
- 한상일
- SCOPUS Author ID
- 한상일
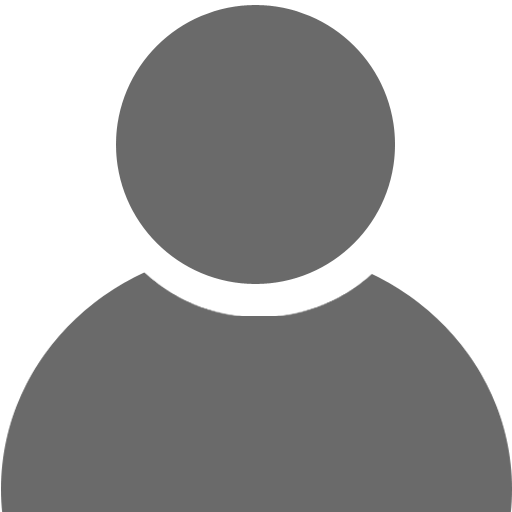
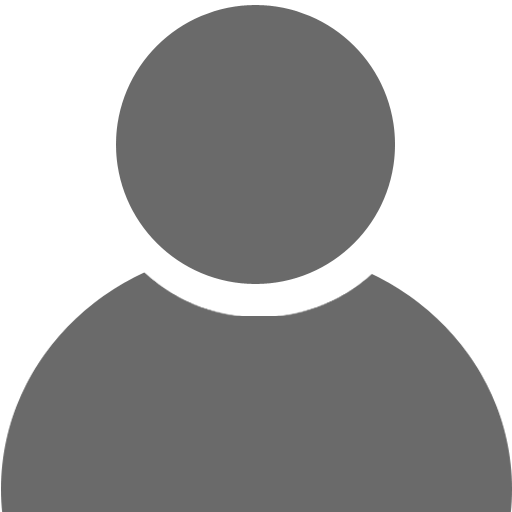
- Issue Date
- 2023
- Journal Title
- Publications of the Astronomical Society of the Pacific
- ISSN
- 4628-6280
- Citation
- Publications of the Astronomical Society of the Pacific vol. 135, no. 1050
- Publisher
- Institute of Physics
- Indexed
- SCIE; SCOPUS

- Document Type
- Article
- Abstract
- We present the construction of an image similarity retrieval engine for the morphological classification of galaxies using the Convolutional AutoEncoder (CAE). The CAE is trained on 90,370 preprocessed Sloan Digital Sky Survey galaxy images listed in the Galaxy Zoo 2 (GZ2) catalog. The visually similar output images returned by the trained CAE suggest that the encoder efficiently compresses input images into latent features, which are then used to calculate similarity parameters. Our Tool for Searching a similar Galaxy Image based on a Convolutional Autoencoder using Similarity (TSGICAS) leverages this similarity parameter to classify galaxies’ morphological types, enabling the identification of a wider range of classes with high accuracy compared to traditional supervised ML techniques. This approach streamlines the researcher’s work by allowing quick prioritization of the most relevant images from the latent feature database. We investigate the accuracy of our automatic morphological classifications using three galaxy catalogs: GZ2, Extraction de Formes Idéalisées de Galaxies en Imagerie (EFIGI), and Nair & Abraham (NA10). The correlation coefficients between the morphological types of input and retrieved galaxy images were found to be 0.735, 0.811, and 0.815 for GZ2, EFIGI, and NA10 catalogs, respectively. Despite differences in morphology tags between input and retrieved galaxy images, visual inspection showed that the two galaxies were very similar, highlighting TSGICAS’s superior performance in image similarity search. We propose that morphological classifications of galaxies using TSGICAS are fast and efficient, making it a valuable tool for detailed galaxy morphological classifications in other imaging surveys. © 2023. The Author(s). Published by IOP Publishing Ltd on behalf of the Astronomical Society of the Pacific (ASP). All rights reserved.
- DOI
- 10.1088/1538-3873/ace851
- Appears in Collections:
- 사범대학 > 과학교육과 > Journal papers
- Files in This Item:
There are no files associated with this item.
- Export
- RIS (EndNote)
- XLS (Excel)
- XML