View : 451 Download: 95
Identification of Major Psychiatric Disorders From Resting-State Electroencephalography Using a Machine Learning Approach
- Title
- Identification of Major Psychiatric Disorders From Resting-State Electroencephalography Using a Machine Learning Approach
- Authors
- Park, Su Mi; Jeong, Boram; Oh, Da Young; Choi, Chi-Hyun; Jung, Hee Yeon; Lee, Jun-Young; Lee, Donghwan; Choi, Jung-Seok
- Ewha Authors
- 이동환
- SCOPUS Author ID
- 이동환
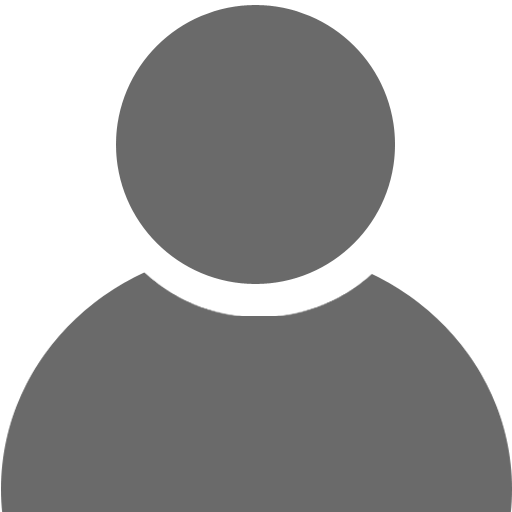
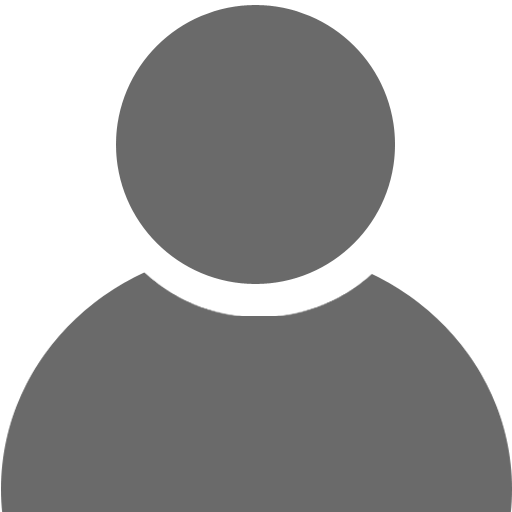
- Issue Date
- 2021
- Journal Title
- FRONTIERS IN PSYCHIATRY
- ISSN
- 1664-0640
- Citation
- FRONTIERS IN PSYCHIATRY vol. 12
- Keywords
- classification; electroencephalography; machine learning; psychiatric disorder; resting-state brain function; power spectrum density; functional connectivity
- Publisher
- FRONTIERS MEDIA SA
- Indexed
- SCIE; SSCI; SCOPUS

- Document Type
- Article
- Abstract
- We aimed to develop a machine learning (ML) classifier to detect and compare major psychiatric disorders using electroencephalography (EEG). We retrospectively collected data from medical records, intelligence quotient (IQ) scores from psychological assessments, and quantitative EEG (QEEG) at resting-state assessments from 945 subjects [850 patients with major psychiatric disorders (six large-categorical and nine specific disorders) and 95 healthy controls (HCs)]. A combination of QEEG parameters including power spectrum density (PSD) and functional connectivity (FC) at frequency bands was used to establish models for the binary classification between patients with each disorder and HCs. The support vector machine, random forest, and elastic net ML methods were applied, and prediction performances were compared. The elastic net model with IQ adjustment showed the highest accuracy. The best feature combinations and classification accuracies for discrimination between patients and HCs with adjusted IQ were as follows: schizophrenia = alpha PSD, 93.83%; trauma and stress-related disorders = beta FC, 91.21%; anxiety disorders = whole band PSD, 91.03%; mood disorders = theta FC, 89.26%; addictive disorders = theta PSD, 85.66%; and obsessive-compulsive disorder = gamma FC, 74.52%. Our findings suggest that ML in EEG may predict major psychiatric disorders and provide an objective index of psychiatric disorders.
- DOI
- 10.3389/fpsyt.2021.707581
- Appears in Collections:
- 자연과학대학 > 통계학전공 > Journal papers
- Files in This Item:
-
fpsyt-12-707581.pdf(1.26 MB)
Download
- Export
- RIS (EndNote)
- XLS (Excel)
- XML