View : 549 Download: 0
Multivariate receptor models and model uncertainty
- Title
- Multivariate receptor models and model uncertainty
- Authors
- Park E.S.; Oh M.-S.; Guttorp P.
- Ewha Authors
- 오만숙
- SCOPUS Author ID
- 오만숙
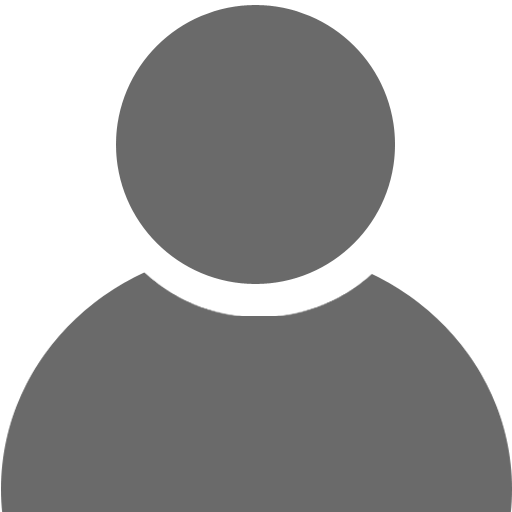
- Issue Date
- 2002
- Journal Title
- Chemometrics and Intelligent Laboratory Systems
- ISSN
- 0169-7439
- Citation
- Chemometrics and Intelligent Laboratory Systems vol. 60, no. 1-2, pp. 49 - 67
- Indexed
- SCI; SCIE; SCOPUS

- Document Type
- Conference Paper
- Abstract
- Estimation of the number of major pollution sources, the source composition profiles, and the source contributions are the main interests in multivariate receptor modeling. Due to lack of identifiability of the receptor model, however, the estimation cannot be done without some additional assumptions. A common approach to this problem is to estimate the number of sources, q, at the first stage, and then estimate source profiles and contributions at the second stage, given additional constraints (identifiability conditions) to prevent source rotation/transformation and the assumption that the q-source model is correct. These assumptions on the parameters (the number of sources and identifiability conditions) are the main source of model uncertainty in multivariate receptor modeling. In this paper, we suggest a Bayesian approach to deal with model uncertainties in multivariate receptor models by using Markov chain Monte Carlo (MCMC) schemes. Specifically, we suggest a method which can simultaneously estimate parameters (compositions and contributions), parameter uncertainties, and model uncertainties (number of sources and identifiability conditions). Simulation results and an application to air pollution data are presented. © 2002 Elsevier Science B.V. All rights reserved.
- DOI
- 10.1016/S0169-7439(01)00185-X
- Appears in Collections:
- 자연과학대학 > 통계학전공 > Journal papers
- Files in This Item:
There are no files associated with this item.
- Export
- RIS (EndNote)
- XLS (Excel)
- XML