View : 562 Download: 0
Robust Bayesian multivariate receptor modeling
- Title
- Robust Bayesian multivariate receptor modeling
- Authors
- Park, Eun Sug; Oh, Man-Suk
- Ewha Authors
- 오만숙
- SCOPUS Author ID
- 오만숙
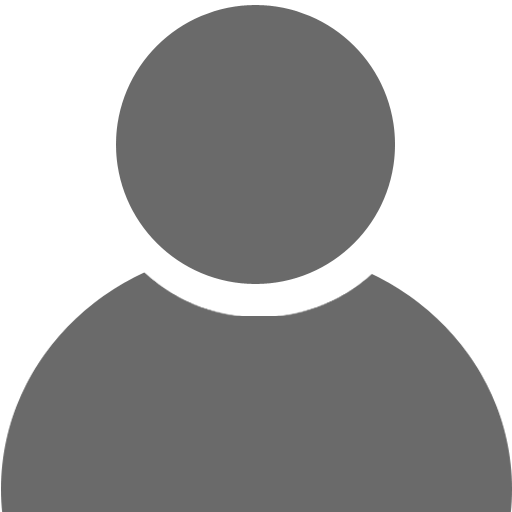
- Issue Date
- 2015
- Journal Title
- CHEMOMETRICS AND INTELLIGENT LABORATORY SYSTEMS
- ISSN
- 0169-7439
1873-3239
- Citation
- CHEMOMETRICS AND INTELLIGENT LABORATORY SYSTEMS vol. 149, pp. 215 - 226
- Keywords
- Source apportionment; Uncertainty estimation; Model uncertainty; Outliers
- Publisher
- ELSEVIER SCIENCE BV
- Indexed
- SCI; SCIE; SCOPUS

- Document Type
- Article
- Abstract
- Multivariate receptor modeling aims to unfold the multivariate air pollution data into components associated with different sources of air pollution based on ambient measurements of air pollutants. It is now a widely accepted approach in source identification and apportionment. An evolving area of research in multivariate receptor modeling is to quantify uncertainty in estimated source contributions as well as model uncertainty caused by the unknown identifiability conditions, sometimes referred to as rotational ambiguity. Unlike the uncertainty estimates for the source composition profiles that have been available in commonly used receptor modeling tools such as positive matrix factorization, little research has been conducted on the uncertainty estimation for the source contributions or the identifiability conditions. Bayesian multivariate receptor modeling based on Markov chain Monte Carol methods is an attractive approach as it offers a great deal of flexibility in both modeling and estimation of parameter uncertainty and model uncertainty. In this paper, we propose a robust Bayesian multivariate receptor modeling approach that can simultaneously estimate uncertainty in source contributions as well as in compositions and uncertainty due to the unknown identifiability conditions by extending the previous Bayesian multivariate receptor modeling in two ways. First, we explicitly account for nonnegativity constraints on the source contributions, in addition to the nonnegativity constraints on the source compositions, in both parameter estimation and model uncertainty estimation. Second, we account for outliers that may often exist in the air pollution data in estimation by considering a heavy-tailed error distribution. The approach is illustrated with both simulated data and real PM2.5 speciation data from Phoenix, Arizona, USA. (C) 2015 Elsevier B.V. All rights reserved.
- DOI
- 10.1016/j.chemolab.2015.08.021
- Appears in Collections:
- 자연과학대학 > 통계학전공 > Journal papers
- Files in This Item:
There are no files associated with this item.
- Export
- RIS (EndNote)
- XLS (Excel)
- XML