View : 90 Download: 0
Multivariate probit linear mixed models for multivariate longitudinal binary data
- Title
- Multivariate probit linear mixed models for multivariate longitudinal binary data
- Authors
- Lee; Kuo-Jung; Kim; Chanmin; Yoo; Jae Keun; Keunbaik
- Ewha Authors
- 유재근
- SCOPUS Author ID
- 유재근
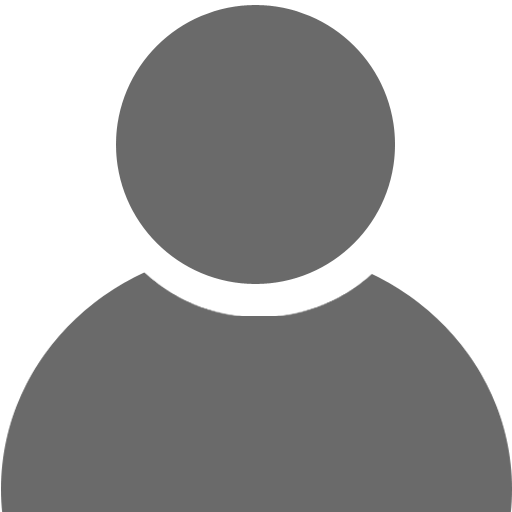
- Issue Date
- 2024
- Journal Title
- Statistics in Medicine
- ISSN
- 0277-6715
- Citation
- Statistics in Medicine vol. 43, no. 8, pp. 1527 - 1548
- Keywords
- correlation matrix; generalized linear mixed models; heterogeneity; hypersphere decomposition; positive definiteness
- Publisher
- John Wiley and Sons Ltd
- Indexed
- SCIE; SCOPUS

- Document Type
- Article
- Abstract
- When analyzing multivariate longitudinal binary data, we estimate the effects on the responses of the covariates while accounting for three types of complex correlations present in the data. These include the correlations within separate responses over time, cross-correlations between different responses at different times, and correlations between different responses at each time point. The number of parameters thus increases quadratically with the dimension of the correlation matrix, making parameter estimation difficult; the estimated correlation matrix must also meet the positive definiteness constraint. The correlation matrix may additionally be heteroscedastic; however, the matrix structure is commonly considered to be homoscedastic and constrained, such as exchangeable or autoregressive with order one. These assumptions are overly strong, resulting in skewed estimates of the covariate effects on the responses. Hence, we propose probit linear mixed models for multivariate longitudinal binary data, where the correlation matrix is estimated using hypersphere decomposition instead of the strong assumptions noted above. Simulations and real examples are used to demonstrate the proposed methods. An open source R package, BayesMGLM, is made available on GitHub at https://github.com/kuojunglee/BayesMGLM/ with full documentation to produce the results. © 2024 John Wiley & Sons Ltd.
- DOI
- 10.1002/sim.10029
- Appears in Collections:
- 자연과학대학 > 통계학전공 > Journal papers
- Files in This Item:
There are no files associated with this item.
- Export
- RIS (EndNote)
- XLS (Excel)
- XML