View : 318 Download: 0
Electrical impedance tomography with deep Calderon method
- Title
- Electrical impedance tomography with deep Calderon method
- Authors
- Cen, Siyu; Jin, Bangti; Shin, Kwancheol; Zhou, Zhi
- Ewha Authors
- 신관철
- SCOPUS Author ID
- 신관철
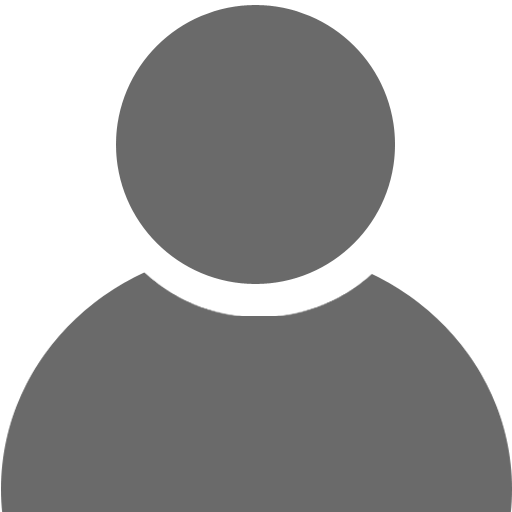
- Issue Date
- 2023
- Journal Title
- JOURNAL OF COMPUTATIONAL PHYSICS
- ISSN
- 0021-9991
1090-2716
- Citation
- JOURNAL OF COMPUTATIONAL PHYSICS vol. 493
- Keywords
- Calderon's method; Electrical impedance tomography; U-net; Deep learning
- Publisher
- ACADEMIC PRESS INC ELSEVIER SCIENCE
- Indexed
- SCIE; SCOPUS

- Document Type
- Article
- Abstract
- Electrical impedance tomography (EIT) is a noninvasive medical imaging modality utilizing the current-density/voltage data measured on the surface of the subject. Calderon's method is a relatively recent EIT imaging algorithm that is non-iterative, fast, and capable of reconstructing complex-valued electric impedances. However, due to the regularization via low-pass filtering and linearization, the reconstructed images suffer from severe blurring and under-estimation of the exact conductivity values. In this work, we develop an enhanced version of Calderon's method, using deep convolution neural networks (i.e., Unet) as an effective targeted post-processing step, and term the resulting method by deep Calderon's method. Specifically, we learn a U-net to postprocess the EIT images generated by Calderon's method so as to have better resolutions and more accurate estimates of conductivity values. We simulate chest configurations with which we generate the currentdensity/voltage boundary measurements and the corresponding reconstructed images by Calderon's method. With the paired training data, we learn the deep neural network and evaluate its performance on real tank measurement data. The experimental results indicate that the proposed approach indeed provides a fast and direct (complex-valued) impedance tomography imaging technique, and substantially improves the capability of the standard Calderon's method.
- DOI
- 10.1016/j.jcp.2023.112427|http://dx.doi.org/10.1016/j.jcp.2023.112427
- Appears in Collections:
- 연구기관 > 수리과학연구소 > Journal papers
- Files in This Item:
There are no files associated with this item.
- Export
- RIS (EndNote)
- XLS (Excel)
- XML