View : 250 Download: 0
Deep Learning Accelerated Design of Mechanically Efficient Architected Materials
- Title
- Deep Learning Accelerated Design of Mechanically Efficient Architected Materials
- Authors
- Lee, Sangryun; Zhang, Zhizhou; Gu, Grace X.
- Ewha Authors
- 이상륜
- SCOPUS Author ID
- 이상륜
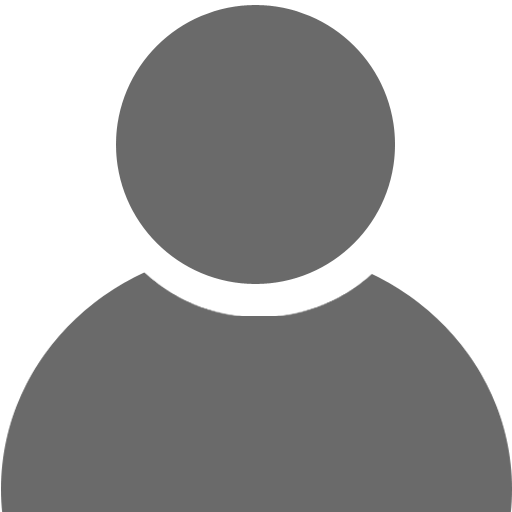
- Issue Date
- 2023
- Journal Title
- ACS APPLIED MATERIALS & INTERFACES
- ISSN
- 1944-8244
1944-8252
- Citation
- ACS APPLIED MATERIALS & INTERFACES vol. 15, no. 18, pp. 22543 - 22552
- Keywords
- lattice structures; deep learning; mechanical properties; additive manufacturing; genetic optimization
- Publisher
- AMER CHEMICAL SOC
- Indexed
- SCIE; SCOPUS

- Document Type
- Article
- Abstract
- Lattice structures are known to have high performance-to-weight ratios because of their highly efficient material distribution in a given volume. However, their inherently large void fraction leads to low mechanical properties compared to the base material, high anisotropy, and brittleness. Most works to date have focused on modifying the spatial arrangement of beam elements to overcome these limitations, but only simple beam geometries are adopted due to the infinitely large design space associated with probing and varying beam shapes. Herein, we present an approach to enhance the elastic modulus, strength, and toughness of lattice structures with minimal tradeoffs by optimizing the shape of beam elements for a suite of lattice structures. A generative deep learning-based approach is employed, which leverages the fast inference of neural networks to accelerate the optimization process. Our optimized lattice structures possess superior stiffness (+59%), strength (+49%), toughness (+106%), and isotropy (+645%) compared to benchmark lattices consisting of cylindrical beams. We fabricate our lattice designs using additive manufacturing to validate the optimization approach; experimental and simulation results show good agreement. Remarkable improvement in mechanical properties is shown to be the effect of distributed stress fields and deformation modes subject to beam shape and lattice type.
- DOI
- 10.1021/acsami.3c02746|http://dx.doi.org/10.1021/acsami.3c02746
- Appears in Collections:
- 공과대학 > 휴먼기계바이오공학과 > Journal papers
- Files in This Item:
There are no files associated with this item.
- Export
- RIS (EndNote)
- XLS (Excel)
- XML