View : 244 Download: 0
Noise-Robust Sleep Staging via Adversarial Training With an Auxiliary Model
- Title
- Noise-Robust Sleep Staging via Adversarial Training With an Auxiliary Model
- Authors
- Yoo, Chaehwa; Liu, Xiaofeng; Xing, Fangxu; El Fakhri, Georges; Woo, Jonghye; Kang, Je-Won
- Ewha Authors
- 강제원
- SCOPUS Author ID
- 강제원
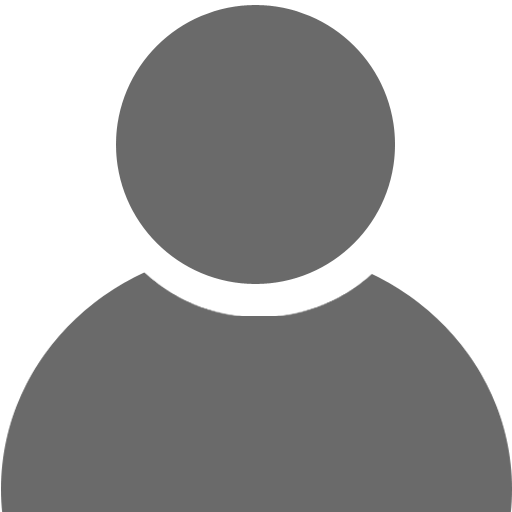
- Issue Date
- 2023
- Journal Title
- IEEE TRANSACTIONS ON BIOMEDICAL ENGINEERING
- ISSN
- 0018-9294
1558-2531
- Citation
- IEEE TRANSACTIONS ON BIOMEDICAL ENGINEERING vol. 70, no. 4, pp. 1252 - 1263
- Keywords
- Adversarial transformation; deep learning; sleep staging; noise-robust neural network
- Publisher
- IEEE-INST ELECTRICAL ELECTRONICS ENGINEERS INC
- Indexed
- SCIE; SCOPUS

- Document Type
- Article
- Abstract
- Deep learning (DL)-based automatic sleep staging approaches have attracted much attention recently due in part to their outstanding accuracy. At the testing stage, however, the performance of these approaches is likely to be degraded, when applied in different testing environments, because of the problem of domain shift. This is because while a pre-trained model is typically trained on noise-free electroencephalogram (EEG) signals acquired from accurate medical equipment, deployment is carried out on consumer-level devices with undesirable noise. To alleviate this challenge, in this work, we propose an efficient training approach that is robust against unseen arbitrary noise. In particular, we propose to generate the worst-case input perturbations by means of adversarial transformation in an auxiliary model, to learn a wide range of input perturbations and thereby to improve reliability. Our approach is based on two separate training models: (i) an auxiliary model to generate adversarial noise and (ii) a target network to incorporate the noise signal to enhance robustness. Furthermore, we exploit novel class-wise robustness during the training of the target network to represent different robustness patterns of each sleep stage. Our experimental results demonstrated that our approach improved sleep staging performance on healthy controls, in the presence of moderate to severe noise levels, compared with competing methods. Our approach was able to effectively train and deploy a DL model to handle different types of noise, including adversarial, Gaussian, and shot noise.
- DOI
- 10.1109/TBME.2022.3214269
- Appears in Collections:
- 공과대학 > 전자전기공학전공 > Journal papers
- Files in This Item:
There are no files associated with this item.
- Export
- RIS (EndNote)
- XLS (Excel)
- XML