View : 358 Download: 0
Performance assessment and optimization of forward osmosis–low pressure ultrafiltration hybrid system using machine learning for rhodamine B removal
- Title
- Performance assessment and optimization of forward osmosis–low pressure ultrafiltration hybrid system using machine learning for rhodamine B removal
- Authors
- Nam S.-N.; Kim S.; Her N.; Choong C.E.; Jang M.; Park C.M.; Heo J.; Yoon Y.
- Ewha Authors
- 윤여민
- SCOPUS Author ID
- 윤여민
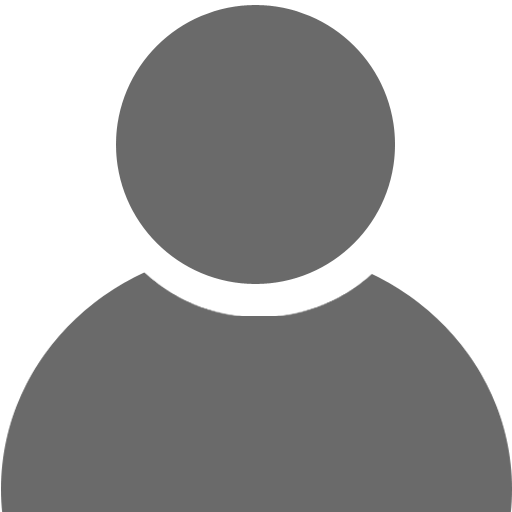
- Issue Date
- 2022
- Journal Title
- Desalination
- ISSN
- 0011-9164
- Citation
- Desalination vol. 543
- Keywords
- Artificial neural network; Forward osmosis; Machine learning; Response surface methodology; Ultrafiltration
- Publisher
- Elsevier B.V.
- Indexed
- SCIE; SCOPUS

- Document Type
- Article
- Abstract
- Here, an in-series forward osmosis-low pressure ultrafiltration membrane system was devised for water treatment. Response surface methodology (RSM) and artificial neural network (ANN) machine learning techniques were applied to evaluate this system's performance and optimization with respect to water flux and rhodamine B (RhB) removal. The effects of initial feed solution (FS) concentration, draw solution (DS) concentration, initial feed pH, and natural organic matter (NOM) concentrate were examined using a central composite design. Models developed using RSM and ANN could successfully fit and predict the data with >99.5 % accuracy. DS and FS concentrations were the most influential factors on water flux and RhB removal, respectively. Statistical parameters demonstrated that ANN approach (water flux = 98.46 % and RhB removal = 99.85 %) was more reliable than RSM; it also better predicted system performance (water flux = 95.06 %; RhB removal = 97.01 %). The predicted optimum conditions for water flux and RhB removal were FS concentration = 47.01 mg L−1, DS concentration = 4.91 M, initial pH = 10.99, and NOM concentration = 7.97 mg L−1. Under these conditions, RSM and ANN models predicted water fluxes of 16.37 L m−2 h−1 and 16.38 L m−2 h−1, respectively, and RhB removal of 99.99 % and 99.95 %, respectively. © 2022
- DOI
- 10.1016/j.desal.2022.116102
- Appears in Collections:
- 공과대학 > 환경공학과 > Journal papers
- Files in This Item:
There are no files associated with this item.
- Export
- RIS (EndNote)
- XLS (Excel)
- XML