View : 692 Download: 0
Unsupervised Black-Box Model Domain Adaptation for Brain Tumor Segmentation
- Title
- Unsupervised Black-Box Model Domain Adaptation for Brain Tumor Segmentation
- Authors
- Liu X.; Yoo C.; Xing F.; Kuo C.C.J.; El Fakhri G.; Kang J.-W.; Woo J.
- Ewha Authors
- 강제원
- SCOPUS Author ID
- 강제원
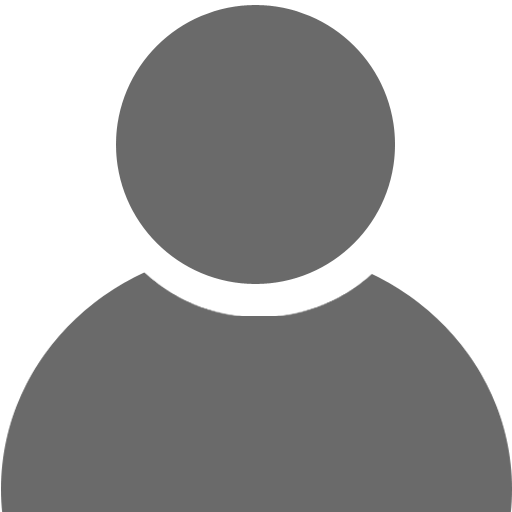
- Issue Date
- 2022
- Journal Title
- Frontiers in Neuroscience
- ISSN
- 1662-4548
- Citation
- Frontiers in Neuroscience vol. 16
- Keywords
- black-box model; brain tumor; knowledge distillation; MR image; segmentation; unsupervised domain adaptation
- Publisher
- Frontiers Media S.A.
- Indexed
- SCIE; SCOPUS

- Document Type
- Article
- Abstract
- Unsupervised domain adaptation (UDA) is an emerging technique that enables the transfer of domain knowledge learned from a labeled source domain to unlabeled target domains, providing a way of coping with the difficulty of labeling in new domains. The majority of prior work has relied on both source and target domain data for adaptation. However, because of privacy concerns about potential leaks in sensitive information contained in patient data, it is often challenging to share the data and labels in the source domain and trained model parameters in cross-center collaborations. To address this issue, we propose a practical framework for UDA with a black-box segmentation model trained in the source domain only, without relying on source data or a white-box source model in which the network parameters are accessible. In particular, we propose a knowledge distillation scheme to gradually learn target-specific representations. Additionally, we regularize the confidence of the labels in the target domain via unsupervised entropy minimization, leading to performance gain over UDA without entropy minimization. We extensively validated our framework on a few datasets and deep learning backbones, demonstrating the potential for our framework to be applied in challenging yet realistic clinical settings. Copyright © 2022 Liu, Yoo, Xing, Kuo, El Fakhri, Kang and Woo.
- DOI
- 10.3389/fnins.2022.837646
- Appears in Collections:
- 공과대학 > 전자전기공학전공 > Journal papers
- Files in This Item:
There are no files associated with this item.
- Export
- RIS (EndNote)
- XLS (Excel)
- XML