View : 521 Download: 0
Robust Change Detection Using Channel-Wise co-Attention-Based Siamese Network With Contrastive Loss Function
- Title
- Robust Change Detection Using Channel-Wise co-Attention-Based Siamese Network With Contrastive Loss Function
- Authors
- Choi E.; Kim J.
- Ewha Authors
- 김정태
- SCOPUS Author ID
- 김정태
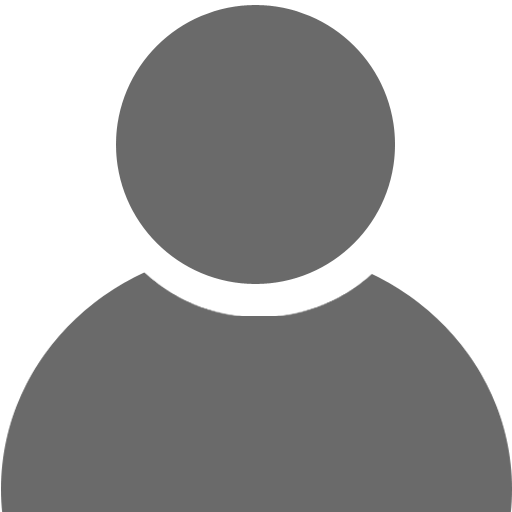
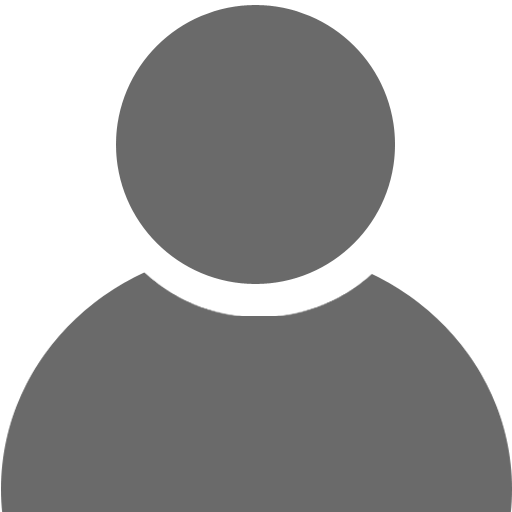
- Issue Date
- 2022
- Journal Title
- IEEE Access
- ISSN
- 2169-3536
- Citation
- IEEE Access vol. 10, pp. 45365 - 45374
- Keywords
- Attention; change detection; co-attention; deep learning; remote sensing; Siamese network
- Publisher
- Institute of Electrical and Electronics Engineers Inc.
- Indexed
- SCIE; SCOPUS

- Document Type
- Article
- Abstract
- Change detection methods aim to identify significantly changed areas in co-registered bitemporal images taken of the same area. Since not only do bitemporal images usually have different environmental conditions (i.e., different weather conditions, noises, and seasonal changes) but also changes irrelevant to the purpose of change detection (e.g., road changes when detecting building change), which should not be detected as changed areas, change detection methods often suffer from the problem of pseudo-change detection. To alleviate this problem, we propose an encoder-decoder-based Siamese network with a channel-wise co-attention module that considers the channel-wise correlations between a feature map in one image and all feature maps in the other image. By comparing the feature map in one image with the revised feature map in the other image considering the correlations, we are able to reduce the differences between the feature maps when pseudo-changes exist, thereby rendering the proposed method more robust to pseudo-changes. In addition, we apply a contrastive loss function that encourages the pairs of feature maps corresponding to unchanged regions to be similar, which can help improve the performance of change detection. We verified the performance of the proposed method through experiments using datasets such as the change detection dataset (CDD) and building change detection dataset (BCDD). In the experiment, the proposed method achieved significantly improved performance compared with existing methods in terms of recall, precision, f1-score, and overall accuracy. © 2013 IEEE.
- DOI
- 10.1109/ACCESS.2022.3170704
- Appears in Collections:
- 공과대학 > 전자전기공학전공 > Journal papers
- Files in This Item:
There are no files associated with this item.
- Export
- RIS (EndNote)
- XLS (Excel)
- XML