View : 544 Download: 0
Purine metabolite-based machine learning models for risk prediction, prognosis, and diagnosis of coronary artery disease
- Title
- Purine metabolite-based machine learning models for risk prediction, prognosis, and diagnosis of coronary artery disease
- Authors
- Jung S.; Ahn E.; Koh S.B.; Lee S.-H.; Hwang G.-S.
- Ewha Authors
- 황금숙
- SCOPUS Author ID
- 황금숙
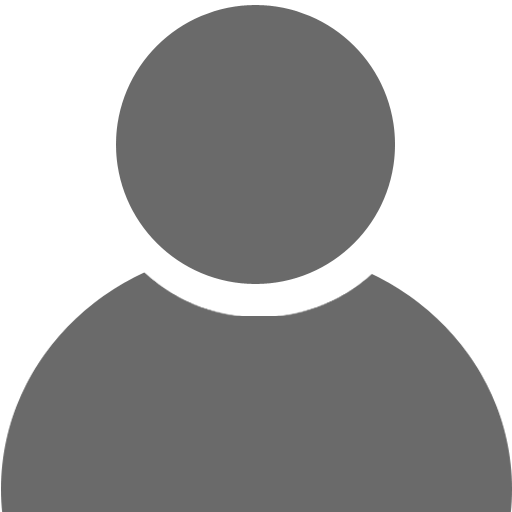
- Issue Date
- 2021
- Journal Title
- Biomedicine and Pharmacotherapy
- ISSN
- 0753-3322
- Citation
- Biomedicine and Pharmacotherapy vol. 139
- Keywords
- Biomarker; Coronary artery disease (CAD); Machine learning model development; Metabolomics; Purine metabolism
- Publisher
- Elsevier Masson s.r.l.
- Indexed
- SCIE; SCOPUS

- Document Type
- Article
- Abstract
- Alterations in xanthine oxidase activity are known to be pathologically influential on coronary artery disease (CAD), but the association between purine-related blood metabolites and CAD has only been partially elucidated. We performed global metabolomics profiling and network analysis on blood samples from the Wonju and Pyeongchang (WP) cohort study (n = 2055) to elucidate the importance of purine related metabolites associated with potential CAD risk. Then, 5 selected serum metabolites were quantified from the WP cohort, Shinchon cohort (n = 259), and Shinchon case control (n = 424) groups to develop machine learning models for 10-year risk prediction, relapse within 10 years and diagnosis of the disease via 100 repeated 5-fold cross-validations of logistic models. The combination of purine metabolite levels or only xanthine levels in blood could be applied for machine learning model development for major adverse cardiac and cerebrovascular event (MACCE, cerebrovascular death, nonfatal myocardial infarction, percutaneous transluminal coronary angioplasty, coronary artery bypass graft, and stroke) risk prediction, relapse of MACCEs among patients with myocardial infarction history and diagnosis of stable CAD. In particular, our research provided initial evidence that blood xanthine and uric acid levels play different roles in the development of machine learning models for primary/secondary prevention or diagnosis of CAD. In this research, we determined that purine-related metabolites in blood are applicable to machine learning model development for CAD risk prediction and diagnosis. Also, our work advances current CAD biomarker discovery strategies mainly relying on clinical features; emphasizes the differential biomarkers in first/secondary prevention or diagnosis studies. © 2021
- DOI
- 10.1016/j.biopha.2021.111621
- Appears in Collections:
- 자연과학대학 > 화학·나노과학전공 > Journal papers
- Files in This Item:
There are no files associated with this item.
- Export
- RIS (EndNote)
- XLS (Excel)
- XML