View : 633 Download: 0
A Real-Time Depth of Anesthesia Monitoring System Based on Deep Neural Network With Large EDO Tolerant EEG Analog Front-End
- Title
- A Real-Time Depth of Anesthesia Monitoring System Based on Deep Neural Network With Large EDO Tolerant EEG Analog Front-End
- Authors
- Park, Yongjae; Han, Su-Hyun; Byun, Wooseok; Kim, Ji-Hoon; Lee, Hyung-Chul; Kim, Seong-Jin
- Ewha Authors
- 김지훈
- SCOPUS Author ID
- 김지훈
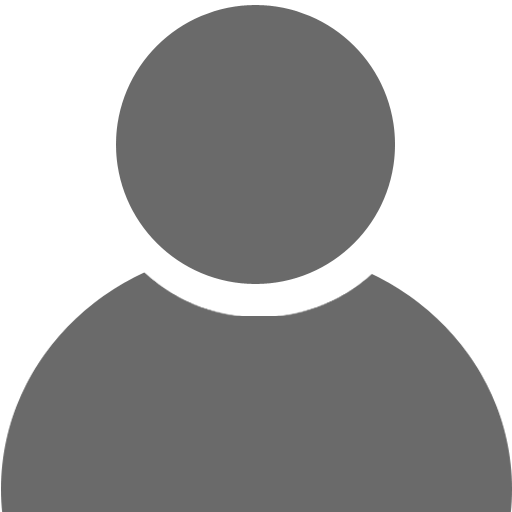
- Issue Date
- 2020
- Journal Title
- IEEE TRANSACTIONS ON BIOMEDICAL CIRCUITS AND SYSTEMS
- ISSN
- 1932-4545
1940-9990
- Citation
- IEEE TRANSACTIONS ON BIOMEDICAL CIRCUITS AND SYSTEMS vol. 14, no. 4, pp. 825 - 837
- Keywords
- Electroencephalography; Monitoring; Anesthesia; DSL; Indexes; Direction-of-arrival estimation; Electrodes; Bispectral index; convolutional neural network; depth of anesthesia monitoring; electrode DC offset; electroencephalogram; latency; minimum alveolar concentration; Raspberry Pi 3
- Publisher
- IEEE-INST ELECTRICAL ELECTRONICS ENGINEERS INC
- Indexed
- SCIE; SCOPUS

- Document Type
- Article
Proceedings Paper
- Abstract
- In this article, we present a real-time electroencephalogram (EEG) based depth of anesthesia (DoA) monitoring system in conjunction with a deep learning framework, AnesNET. An EEG analog front-end (AFE) that can compensate +/- 380-mV electrode DC offset using a coarse digital DC servo loop is implemented in the proposed system. The EEG-based MAC, EEGMAC, is introduced as a novel index to accurately predict the DoA, which is designed for applying to patients anesthetized by both volatile and intravenous agents. The proposed deep learning protocol consists of four layers of convolutional neural network and two dense layers. In addition, we optimize the complexity of the deep neural network (DNN) to operate on a microcomputer such as the Raspberry Pi 3, realizing a cost-effective small-size DoA monitoring system. Fabricated in 110-nm CMOS, the prototype AFE consumes 4.33 mu W per channel and has the input-referred noise of 0.29 mu Vrms from 0.5 to 100 Hz with the noise efficiency factor of 2.2. The proposed DNN was evaluated with pre-recorded EEG data from 374 subjects administrated by inhalational anesthetics under surgery, achieving an average squared and absolute errors of 0.048 and 0.05, respectively. The EEGMAC with subjects anesthetized by an intravenous agent also showed a good agreement with the bispectral index value, confirming the proposed DoA index is applicable to both anesthetics. The implemented monitoring system with the Raspberry Pi 3 estimates the EEGMAC within 20 ms, which is about thousand-fold faster than the BIS estimation in literature.
- DOI
- 10.1109/TBCAS.2020.2998172
- Appears in Collections:
- 공과대학 > 전자전기공학전공 > Journal papers
- Files in This Item:
There are no files associated with this item.
- Export
- RIS (EndNote)
- XLS (Excel)
- XML