View : 732 Download: 0
Bayesian analysis of financial volatilities addressing long-memory, conditional heteroscedasticity and skewed error distribution
- Title
- Bayesian analysis of financial volatilities addressing long-memory, conditional heteroscedasticity and skewed error distribution
- Authors
- Oh R.; Shin D.W.; Oh M.-S.
- Ewha Authors
- 오만숙; 신동완
- SCOPUS Author ID
- 오만숙
; 신동완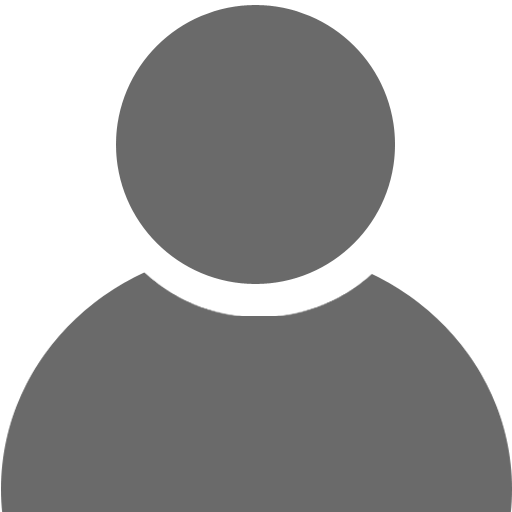
- Issue Date
- 2017
- Journal Title
- Communications for Statistical Applications and Methods
- ISSN
- 2287-7843
- Citation
- Communications for Statistical Applications and Methods vol. 24, no. 5, pp. 507 - 518
- Keywords
- ARFIMA; Bayesian; GARCH; JAGS; Markov chain Monte Carlo; Skewed-t
- Publisher
- Korean Statistical Society
- Indexed
- SCOPUS; KCI

- Document Type
- Article
- Abstract
- Volatility plays a crucial role in theory and applications of asset pricing, optimal portfolio allocation, and risk management. This paper proposes a combined model of autoregressive moving average (ARFIMA), generalized autoregressive conditional heteroscedasticity (GRACH), and skewed-t error distribution to accommodate important features of volatility data; long memory, heteroscedasticity, and asymmetric error distribution. A fully Bayesian approach is proposed to estimate the parameters of the model simultaneously, which yields parameter estimates satisfying necessary constraints in the model. The approach can be easily implemented using a free and user-friendly software JAGS to generate Markov chain Monte Carlo samples from the joint posterior distribution of the parameters. The method is illustrated by using a daily volatility index from Chicago Board Options Exchange (CBOE). JAGS codes for model specification is provided in the Appendix. © 2017 The Korean Statistical Society, and Korean International Statistical Society.
- DOI
- 10.5351/CSAM.2017.24.5.507
- Appears in Collections:
- 자연과학대학 > 통계학전공 > Journal papers
- Files in This Item:
There are no files associated with this item.
- Export
- RIS (EndNote)
- XLS (Excel)
- XML