View : 607 Download: 0
Partial least squares fusing unsupervised learning
- Title
- Partial least squares fusing unsupervised learning
- Authors
- Yoo J.K.
- Ewha Authors
- 유재근
- SCOPUS Author ID
- 유재근
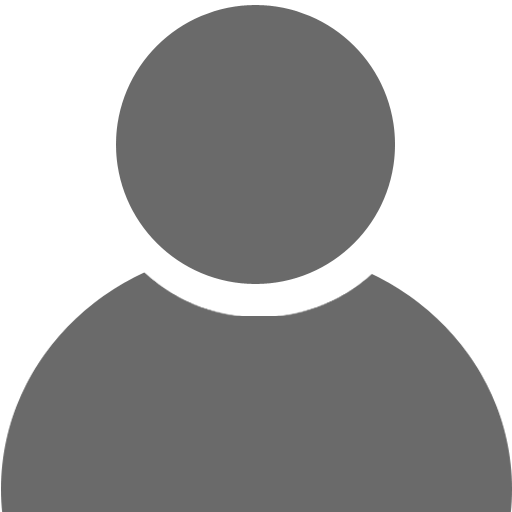
- Issue Date
- 2018
- Journal Title
- Chemometrics and Intelligent Laboratory Systems
- ISSN
- 0169-7439
- Citation
- Chemometrics and Intelligent Laboratory Systems vol. 175, pp. 82 - 86
- Keywords
- Cluster analysis; Fused approach; Large p small n; Multivariate analysis; Partial least squares; Unsupervised learning
- Publisher
- Elsevier B.V.
- Indexed
- SCIE; SCOPUS

- Document Type
- Article
- Abstract
- In this paper, partial least squares to fuse unsupervised learning, called fused clustered least squares (FCLS), is proposed. As an unsupervised method, the K-means clustering algorithm is adopted, and it clusters either the original predictors or its principal components. This unsupervised learning procedure has a function to discover unknown structures of the predictors, and this information is utilized in their further reduction. Within each cluster, the covariance of the response and the predictors is computed and successively projected onto the covariance matrix of the predictors. This is called clustered least squares. Then we fuse all clustered least squares from the various numbers of clusters. The FCLS is basically implemented by combining supervised and unsupervised statistical methods, and it overcomes the deficits that the ordinary least squares, including its popular variation of partial least squares, have in practice. Numerical studies support the theory, and its application to near infrared spectroscopy data confirms the potential advantage of FCLS in practice. © 2018 Elsevier B.V.
- DOI
- 10.1016/j.chemolab.2017.12.016
- Appears in Collections:
- 자연과학대학 > 통계학전공 > Journal papers
- Files in This Item:
There are no files associated with this item.
- Export
- RIS (EndNote)
- XLS (Excel)
- XML