View : 632 Download: 0
On multivariate discrete least squares
- Title
- On multivariate discrete least squares
- Authors
- Lee Y.J.; Micchelli C.A.; Yoon J.
- Ewha Authors
- 윤정호
- SCOPUS Author ID
- 윤정호
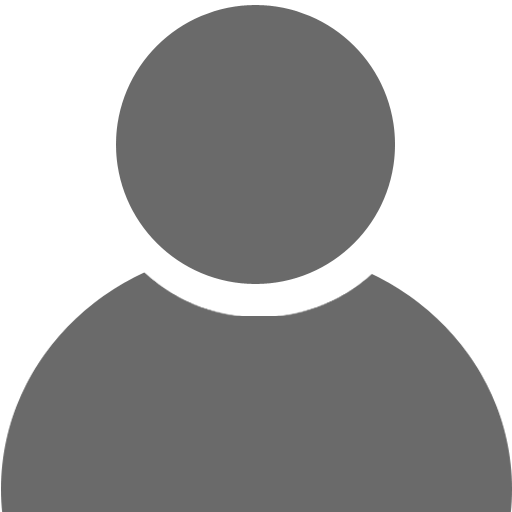
- Issue Date
- 2016
- Journal Title
- Journal of Approximation Theory
- ISSN
- 0021-9045
- Citation
- Journal of Approximation Theory vol. 211, pp. 78 - 84
- Keywords
- Collocation matrix; Multivariate least squares; Multivariate Maclaurin expansion; Wronskian
- Publisher
- Academic Press Inc.
- Indexed
- SCIE; SCOPUS

- Document Type
- Article
- Abstract
- For a positive integer n∈N we introduce the index set Nn:={1,2,…,n}. Let X:={xi:i∈Nn} be a distinct set of vectors in Rd, Y:={yi:i∈Nn} a prescribed data set of real numbers in R and F:={fj:j∈Nm},m<n, a given set of real valued continuous functions defined on some neighborhood O of Rd containing X. The discrete least squares problem determines a (generally unique) function f=∑j∈Nmcj ⋆fj∈spanF which minimizes the square of the ℓ2−norm ∑i∈Nn(∑j∈Nmcjfj(xi)−yi)2 over all vectors (cj:j∈Nm)∈Rm. The value of f at some s∈O may be viewed as the optimally predicted value (in the ℓ2−sense) of all functions in spanF from the given data X={xi:i∈Nn} and Y={yi:i∈Nn}. We ask “What happens if the components of X and s are nearly the same”. For example, when all these vectors are near the origin in Rd. From a practical point of view this problem comes up in image analysis when we wish to obtain a new pixel value from nearby available pixel values as was done in [2], for a specified set of functions F. This problem was satisfactorily solved in the univariate case in Section 6 of Lee and Micchelli (2013). Here, we treat the significantly more difficult multivariate case using an approach recently provided in Yeon Ju Lee, Charles A. Micchelli and Jungho Yoon (2015). © 2016
- DOI
- 10.1016/j.jat.2016.07.005
- Appears in Collections:
- 자연과학대학 > 수학전공 > Journal papers
- Files in This Item:
There are no files associated with this item.
- Export
- RIS (EndNote)
- XLS (Excel)
- XML