View : 703 Download: 0
Online learning in BitTorrent systems
- Title
- Online learning in BitTorrent systems
- Authors
- Izhak-Ratzin R.; Park H.; Schaar M.V.D.
- Ewha Authors
- 박형곤
- SCOPUS Author ID
- 박형곤
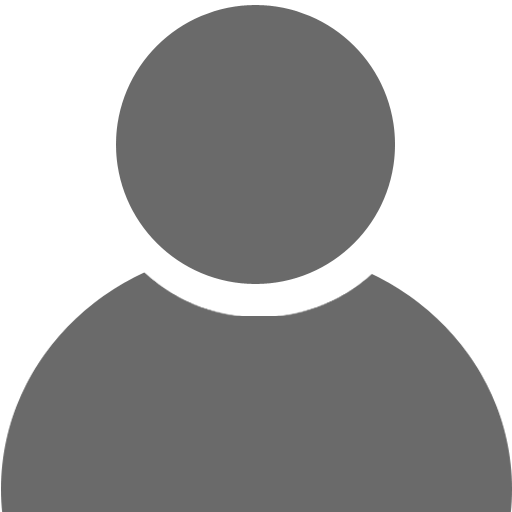
- Issue Date
- 2012
- Journal Title
- IEEE Transactions on Parallel and Distributed Systems
- ISSN
- 1045-9219
- Citation
- IEEE Transactions on Parallel and Distributed Systems vol. 23, no. 12, pp. 2280 - 2288
- Indexed
- SCI; SCIE; SCOPUS

- Document Type
- Article
- Abstract
- We propose a BitTorrent-like protocol based on an online learning (reinforcement learning) mechanism, which can replace the peer selection mechanisms in the regular BitTorrent protocol. We model the peers' interactions in the BitTorrent-like network as a repeated stochastic game, where the strategic behaviors of the peers are explicitly considered. A peer that applies the reinforcement learning (RL)-based mechanism uses the observations on the associated peers' statistical reciprocal behaviors to determine its best responses and estimate the corresponding impact on its expected utility. The policy determines the peer's resource reciprocations such that the peer can maximize its long-term performance. We have implemented the proposed mechanism and incorporated it into an existing BitTorrent client. Our experiments performed on a controlled Planetlab testbed confirm that the proposed protocol 1) promotes fairness and provides incentives to contributed resources, i.e., high capacity peers improve their download completion time by up to 33 percent, 2) improves the system stability and robustness, i.e., reduces the peer selection fluctuations by 57 percent, and (3) discourages free-riding, i.e., peers reduce their uploads to free-riders by 64 percent as compared to the regular BitTorrent protocol. © 1990-2012 IEEE.
- DOI
- 10.1109/TPDS.2012.90
- Appears in Collections:
- 공과대학 > 전자전기공학전공 > Journal papers
- Files in This Item:
There are no files associated with this item.
- Export
- RIS (EndNote)
- XLS (Excel)
- XML