View : 44 Download: 0
Skin Lesion Classification Using a Deep Ensemble Model
- Title
- Skin Lesion Classification Using a Deep Ensemble Model
- Authors
- Thwin; Su Myat; Park; Hyun-Seok
- Ewha Authors
- 박현석
- SCOPUS Author ID
- 박현석
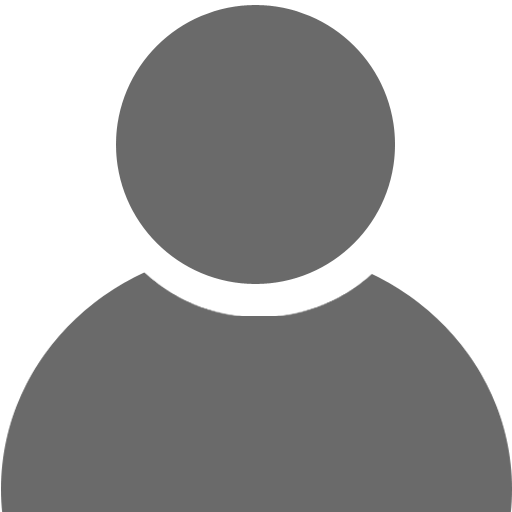
- Issue Date
- 2024
- Journal Title
- Applied Sciences (Switzerland)
- ISSN
- 2076-3417
- Citation
- Applied Sciences (Switzerland) vol. 14, no. 13
- Keywords
- ensemble; Inception-V3; ResNet-50; skin lesion classification; VGG16
- Indexed
- SCIE; SCOPUS

- Document Type
- Article
- Abstract
- Skin cancer, particularly melanoma, is a severe health threat that necessitates early detection for effective treatment. This research introduces a skin lesion classification system that harnesses the capabilities of three advanced deep learning models: VGG16, Inception-V3, and ResNet-50. By integrating these models into an ensemble, the system leverages their individual strengths to improve classification accuracy and robustness. Every model in the ensemble brings its distinctive contribution, having undergone pre-training on ImageNet and subsequent fine-tuning using dermoscopic images. We evaluated our ensemble approach using the ISIC 2018 dataset, a standard benchmark for skin lesion classification. By employing a weighted averaging method to merge predictions from the three models, our ensemble model demonstrated superior performance. The results show an improvement in classification accuracy, achieving an accuracy of 91% on the original dataset and 97% on the dataset balanced by oversampling compared to the individual models. This system was then evaluated using the HAM10000 dataset. The results using the HAM10000 dataset show an improvement in classification accuracy, achieving an accuracy of 90% on the original dataset and 96% on the dataset balanced by oversampling compared to the individual models. This enhanced performance highlights the effectiveness of our ensemble method in capturing diverse features of skin lesions, leading to more accurate diagnoses. Our findings suggest that this approach can significantly assist dermatologists in early and precise skin cancer detection, potentially improving patient outcomes. © 2024 by the authors.
- DOI
- 10.3390/app14135599
- Appears in Collections:
- 인공지능대학 > 컴퓨터공학과 > Journal papers
- Files in This Item:
There are no files associated with this item.
- Export
- RIS (EndNote)
- XLS (Excel)
- XML