View : 229 Download: 0
Machine Learning-assisted Quantitative Mapping of Intracortical Axonal Plasticity Following a Focal Cortical Stroke in Rodents
- Title
- Machine Learning-assisted Quantitative Mapping of Intracortical Axonal Plasticity Following a Focal Cortical Stroke in Rodents
- Authors
- Kim, Hyung Soon; Seo, Hyo Gyeong; Jhee, Jong Ho; Park, Chang Hyun; Lee, Hyang Woon; Park, Bumhee; Kim, Byung Gon
- Ewha Authors
- 이향운; 박창현
- SCOPUS Author ID
- 이향운
; 박창현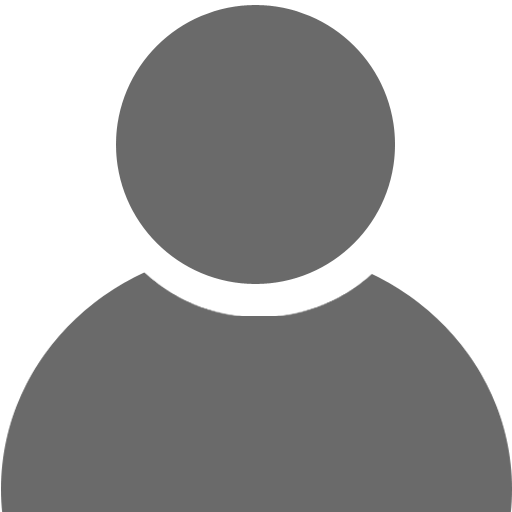
- Issue Date
- 2023
- Journal Title
- EXPERIMENTAL NEUROBIOLOGY
- ISSN
- 1226-2560
2093-8144
- Citation
- EXPERIMENTAL NEUROBIOLOGY vol. 32, no. 3, pp. 170 - 180
- Keywords
- Ischemic stroke; Motor cortex; Neuronal plasticity; Machine learning; Support vector machine; Neuroanatomical tract-tracing techniques
- Publisher
- KOREAN SOC BRAIN &
NEURAL SCIENCE, KOREAN SOC NEURODEGENERATIVE DISEASE
- Indexed
- SCIE; SCOPUS; KCI

- Document Type
- Article
- Abstract
- Stroke destroys neurons and their connections leading to focal neurological deficits. Although limited, many patients exhibit a certain degree of spontaneous functional recovery. Structural remodeling of the intracortical axonal connections is implicated in the reorganization of cortical motor representation maps, which is considered to be an underlying mechanism of the improvement in motor function. Therefore, an accurate assessment of intracortical axonal plasticity would be necessary to develop strategies to facilitate functional recovery following a stroke. The present study developed a machine learning-assisted image analysis tool based on multi-voxel pattern analysis in fMRI imaging. Intracortical axons originating from the rostral forelimb area (RFA) were anterogradely traced using biotinylated dextran amine (BDA) following a photothrombotic stroke in the mouse motor cortex. BDA-traced axons were visualized in tangentially sectioned cortical tissues, digitally marked, and converted to pixelated axon density maps. Application of the machine learning algorithm enabled sensitive comparison of the quantitative differences and the precise spatial mapping of the post-stroke axonal reorganization even in the regions with dense axonal projections. Using this method, we observed a substantial extent of the axonal sprouting from the RFA to the premotor cortex and the peri-infarct region caudal to the RFA. Therefore, the machine learning assisted quantitative axonal mapping developed in this study can be utilized to discover intracortical axonal plasticity that may mediate functional restoration following stroke.
- DOI
- 10.5607/en23016
- Appears in Collections:
- 의과대학 > 의학과 > Journal papers
- Files in This Item:
There are no files associated with this item.
- Export
- RIS (EndNote)
- XLS (Excel)
- XML