View : 193 Download: 0
Clinical applicability of an artificial intelligence prediction algorithm for early prediction of non-persistent atrial fibrillation
- Title
- Clinical applicability of an artificial intelligence prediction algorithm for early prediction of non-persistent atrial fibrillation
- Authors
- Kim, Yeji; Joo, Gihun; Jeon, Bo-Kyung; Kim, Dong-Hyeok; Shin, Tae Young; Im, Hyeonseung; Park, Junbeom
- Ewha Authors
- 박준범; 김동혁
- SCOPUS Author ID
- 박준범
; 김동혁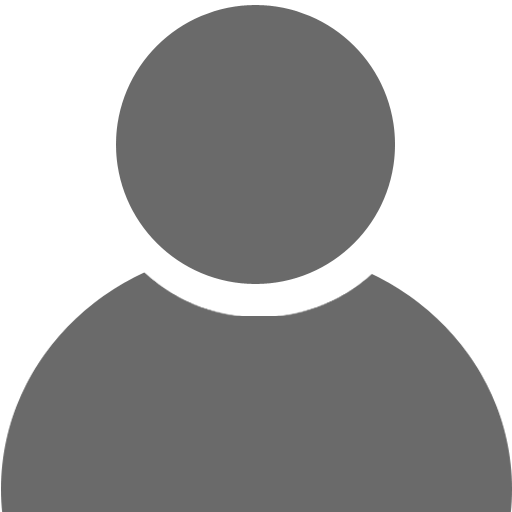
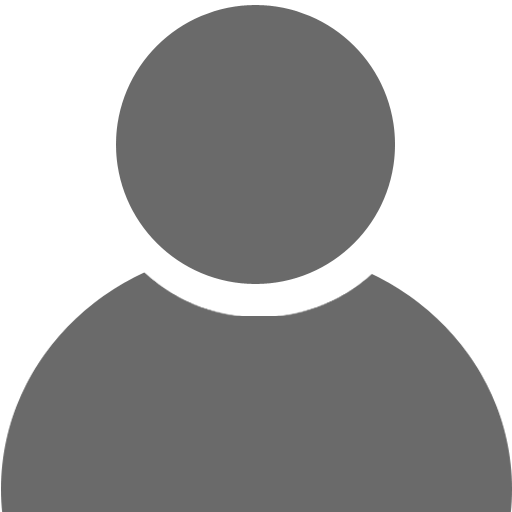
- Issue Date
- 2023
- Journal Title
- FRONTIERS IN CARDIOVASCULAR MEDICINE
- ISSN
- 2297-055X
- Citation
- FRONTIERS IN CARDIOVASCULAR MEDICINE vol. 10
- Keywords
- non-persistent atrial fibrillation; artificial intelligence; convolutional neural network; electrocardiogram; normal sinus rhythm
- Publisher
- FRONTIERS MEDIA SA
- Indexed
- SCIE; SCOPUS

- Document Type
- Article
- Abstract
- Background and aimsIt is difficult to document atrial fibrillation (AF) on ECG in patients with non-persistent atrial fibrillation (non-PeAF). There is limited understanding of whether an AI prediction algorithm could predict the occurrence of non-PeAF from the information of normal sinus rhythm (SR) of a 12-lead ECG. This study aimed to derive a precise predictive AI model for screening non-PeAF using SR ECG within 4 weeks.MethodsThis retrospective cohort study included patients aged 18 to 99 with SR ECG on 12-lead standard ECG (10 seconds) in Ewha Womans University Medical Center for 3 years. Data were preprocessed into three window periods (which are defined with the duration from SR to non-PeAF detection) - 1 week, 2 weeks, and 4 weeks from the AF detection prospectively. For experiments, we adopted a Residual Neural Network model based on 1D-CNN proposed in a previous study. We used 7,595 SR ECGs (extracted from 215,875 ECGs) with window periods of 1 week, 2 weeks, and 4 weeks for analysis.ResultsThe prediction algorithm showed an AUC of 0.862 and an F1-score of 0.84 in the 1:4 matched group of a 1-week window period. For the 1:4 matched group of a 2-week window period, it showed an AUC of 0.864 and an F1-score of 0.85. Finally, for the 1:4 matched group of a 4-week window period, it showed an AUC of 0.842 and an F1-score of 0.83.ConclusionThe AI prediction algorithm showed the possibility of risk stratification for early detection of non-PeAF. Moreover, this study showed that a short window period is also sufficient to detect non-PeAF.
- DOI
- 10.3389/fcvm.2023.1168054
- Appears in Collections:
- 의과대학 > 의학과 > Journal papers
- Files in This Item:
There are no files associated with this item.
- Export
- RIS (EndNote)
- XLS (Excel)
- XML