View : 303 Download: 0
Predicting outcomes of continuous renal replacement therapy using body composition monitoring: a deep-learning approach
- Title
- Predicting outcomes of continuous renal replacement therapy using body composition monitoring: a deep-learning approach
- Authors
- Yoo; Kyung Don; Noh; Junhyug; Bae; Wonho; An; Jung Nam; Oh; Hyung Jung; Rhee; Harin; Seong; Eun Young; Baek; Seon Ha; Ahn; Shin Young; Cho; Jang-Hee; Kim; Dong Ki; Ryu; Dong-Ryeol; Sejoong; Lim; Chun Soo; Lee; Jung Pyo; Sung Gyun; Ko; Gang Jee; Park; Jung Tak; Chang; Tae Ik; Chung; Sungjin; Sang Ho; Choi; Bum Soon; Jeon; Jin Seok; Song; Sangheon; Dae Eun; Jung; Woo Kyung
- Ewha Authors
- 류동열; 노준혁
- SCOPUS Author ID
- 류동열
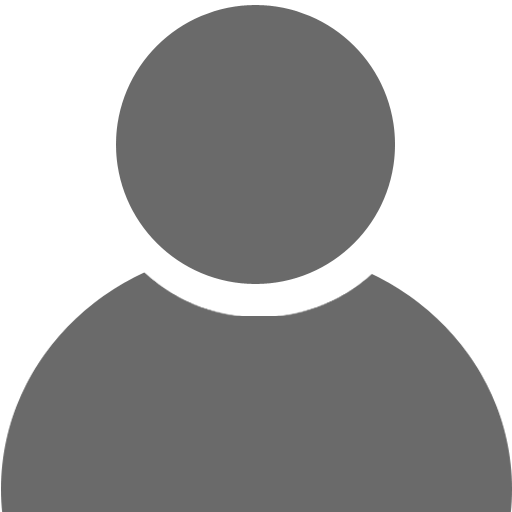
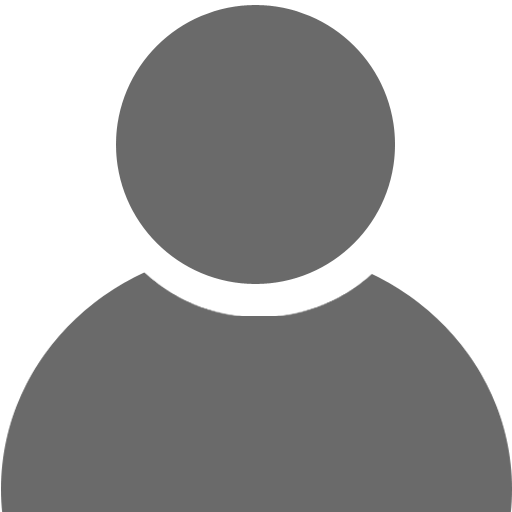
; 노준혁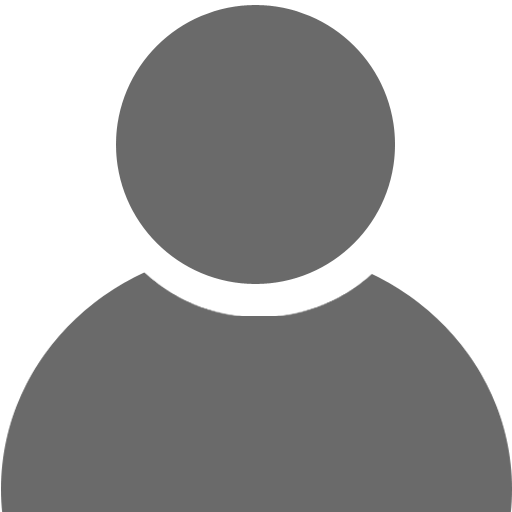
- Issue Date
- 2023
- Journal Title
- Scientific Reports
- ISSN
- 2045-2322
- Citation
- Scientific Reports vol. 13, no. 1
- Publisher
- Nature Research
- Indexed
- SCIE; SCOPUS

- Document Type
- Article
- Abstract
- Fluid balance is a critical prognostic factor for patients with severe acute kidney injury (AKI) requiring continuous renal replacement therapy (CRRT). This study evaluated whether repeated fluid balance monitoring could improve prognosis in this clinical population. This was a multicenter retrospective study that included 784 patients (mean age, 67.8 years; males, 66.4%) with severe AKI requiring CRRT during 2017–2019 who were treated in eight tertiary hospitals in Korea. Sequential changes in total body water were compared between patients who died (event group) and those who survived (control group) using mixed-effects linear regression analyses. The performance of various machine learning methods, including recurrent neural networks, was compared to that of existing prognostic clinical scores. After adjusting for confounding factors, a marginal benefit of fluid balance was identified for the control group compared to that for the event group (p = 0.074). The deep-learning model using a recurrent neural network with an autoencoder and including fluid balance monitoring provided the best differentiation between the groups (area under the curve, 0.793) compared to 0.604 and 0.606 for SOFA and APACHE II scores, respectively. Our prognostic, deep-learning model underlines the importance of fluid balance monitoring for prognosis assessment among patients receiving CRRT. © 2023, The Author(s).
- DOI
- 10.1038/s41598-023-30074-4
- Appears in Collections:
- 의과대학 > 의학과 > Journal papers
- Files in This Item:
There are no files associated with this item.
- Export
- RIS (EndNote)
- XLS (Excel)
- XML