View : 469 Download: 138
Object detectors involving a NAS-gate convolutional module and capsule attention module
- Title
- Object detectors involving a NAS-gate convolutional module and capsule attention module
- Authors
- Viriyasaranon T.; Choi J.-H.
- Ewha Authors
- 최장환
- SCOPUS Author ID
- 최장환
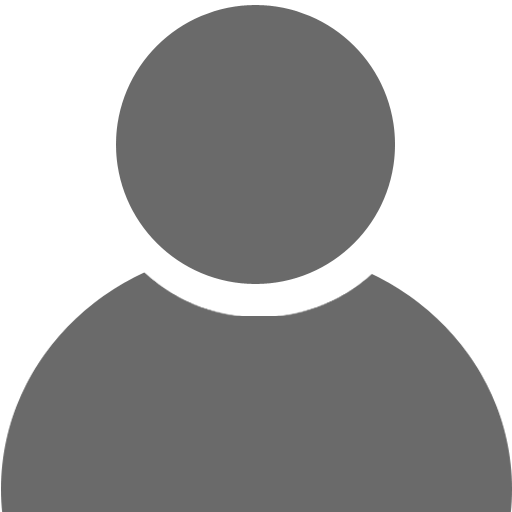
- Issue Date
- 2022
- Journal Title
- Scientific Reports
- ISSN
- 2045-2322
- Citation
- Scientific Reports vol. 12, no. 1
- Publisher
- Nature Research
- Indexed
- SCIE; SCOPUS

- Document Type
- Article
- Abstract
- Several state-of-the-art object detectors have demonstrated outstanding performances by optimizing feature representation through modification of the backbone architecture and exploitation of a feature pyramid. To determine the effectiveness of this approach, we explore the modification of object detectors’ backbone and feature pyramid by utilizing Neural Architecture Search (NAS) and Capsule Network. We introduce two modules, namely, NAS-gate convolutional module and Capsule Attention module. The NAS-gate convolutional module optimizes standard convolution in a backbone network based on differentiable architecture search cooperation with multiple convolution conditions to overcome object scale variation problems. The Capsule Attention module exploits the strong spatial relationship encoding ability of the capsule network to generate a spatial attention mask, which emphasizes important features and suppresses unnecessary features in the feature pyramid, in order to optimize the feature representation and localization capability of the detectors. Experimental results indicate that the NAS-gate convolutional module can alleviate the object scale variation problem and the Capsule Attention network can help to avoid inaccurate localization. Next, we introduce NASGC-CapANet, which incorporates the two modules, i.e., a NAS-gate convolutional module and capsule attention module. Results of comparisons against state-of-the-art object detectors on the MS COCO val-2017 dataset demonstrate that NASGC-CapANet-based Faster R-CNN significantly outperforms the baseline Faster R-CNN with a ResNet-50 backbone and a ResNet-101 backbone by mAPs of 2.7% and 2.0%, respectively. Furthermore, the NASGC-CapANet-based Cascade R-CNN achieves a box mAP of 43.8% on the MS COCO test-dev dataset. © 2022, The Author(s).
- DOI
- 10.1038/s41598-022-07898-7
- Appears in Collections:
- 인공지능대학 > 인공지능학과 > Journal papers
- Files in This Item:
-
s41598-022-07898-7.pdf(2.23 MB)
Download
- Export
- RIS (EndNote)
- XLS (Excel)
- XML