View : 581 Download: 0
Physics-informed deep learning for data-driven solutions of computational fluid dynamics
- Title
- Physics-informed deep learning for data-driven solutions of computational fluid dynamics
- Authors
- Choi S.; Jung I.; Kim H.; Na J.; Lee J.M.
- Ewha Authors
- 나종걸
- SCOPUS Author ID
- 나종걸
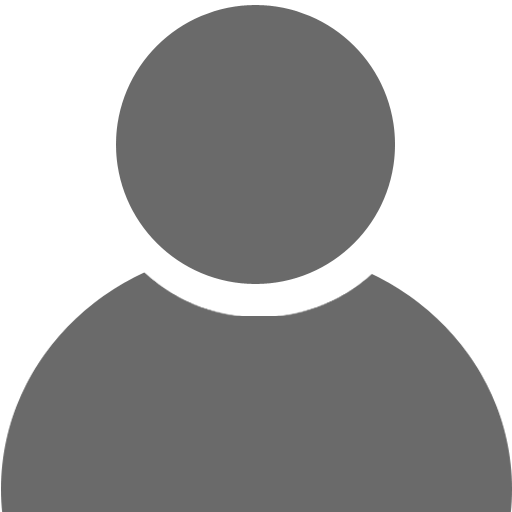
- Issue Date
- 2022
- Journal Title
- Korean Journal of Chemical Engineering
- ISSN
- 0256-1115
- Citation
- Korean Journal of Chemical Engineering vol. 39, no. 3, pp. 515 - 528
- Keywords
- Chemical Reactor; Computational Fluid Dynamics; Physics-informed Neural Network; Surrogate Model
- Publisher
- Springer
- Indexed
- SCIE; SCOPUS; KCI

- Document Type
- Article
- Abstract
- Computational fluid dynamics (CFD) is an essential tool for solving engineering problems that involve fluid dynamics. Especially in chemical engineering, fluid motion usually has extensive effects on system states, such as temperature and component concentration. However, due to the critical issue of long computational times for simulating CFD, application of CFD is limited for many real-time problems, such as real-time optimization and process control. In this study, we developed a surrogate model of a continuous stirred tank reactor (CSTR) with van de Vusse reaction using physics-informed neural network (PINN), which can train the governing equations of the system. We propose a PINN architecture that can train every governing equation which a chemical reactor system follows and can train a multi-reference frame system. Also, we investigated that PINN can resolve the problem of neural network that needs a large number of training data, is easily overfitted and cannot contain physical meaning. Furthermore, we modified the original PINN suggested by Raissi to solve the memory error and divergence problem with two methods: Mini-batch training and weighted loss function. We also suggest a similarity-based sampling strategy where the accuracy can be improved up to five times over random sampling. This work can provide a guideline for developing a high performance surrogate model of the chemical process. © 2022, The Korean Institute of Chemical Engineers.
- DOI
- 10.1007/s11814-021-0979-x
- Appears in Collections:
- 공과대학 > 화공신소재공학과 > Journal papers
- Files in This Item:
There are no files associated with this item.
- Export
- RIS (EndNote)
- XLS (Excel)
- XML