View : 483 Download: 0
An Energy-Efficient Deep Convolutional Neural Network Inference Processor With Enhanced Output Stationary Dataflow in 65-nm CMOS
- Title
- An Energy-Efficient Deep Convolutional Neural Network Inference Processor With Enhanced Output Stationary Dataflow in 65-nm CMOS
- Authors
- Sim, Jaehyeong; Lee, Somin; Kim, Lee-Sup
- Ewha Authors
- 심재형
- SCOPUS Author ID
- 심재형
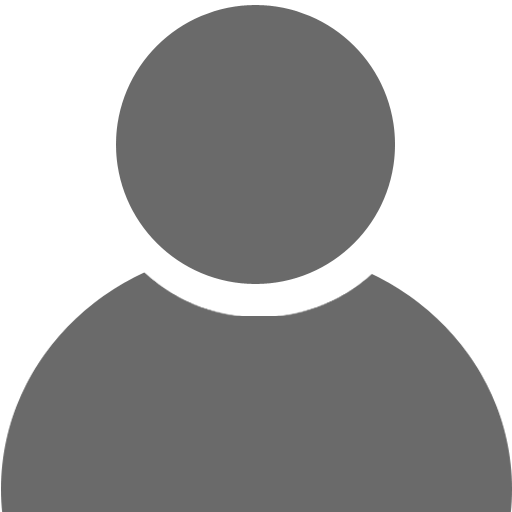
- Issue Date
- 2020
- Journal Title
- IEEE TRANSACTIONS ON VERY LARGE SCALE INTEGRATION (VLSI) SYSTEMS
- ISSN
- 1063-8210
1557-9999
- Citation
- IEEE TRANSACTIONS ON VERY LARGE SCALE INTEGRATION (VLSI) SYSTEMS vol. 28, no. 1, pp. 87 - 100
- Keywords
- Earth Observing System; Radio frequency; Energy consumption; System-on-chip; Memory management; Registers; Random access memory; Convolutional neural network (CNN); dataflow; deep learning; energy-efficient processor; near-threshold voltage (NTV)
- Publisher
- IEEE-INST ELECTRICAL ELECTRONICS ENGINEERS INC
- Indexed
- SCIE; SCOPUS

- Document Type
- Article
- Abstract
- We propose a deep convolutional neural network (CNN) inference processor based on a novel enhanced output stationary (EOS) dataflow. Based on the observation that some activations are commonly used in two successive convolutions, the EOS dataflow employs dedicated register files (RFs) for storing such reused activation data to eliminate redundant memory accesses for highly energy-consuming SRAM banks. In addition, processing elements (PEs) are split into multiple small groups such that each group covers a tile of input activation map to increase the usability of activation RFs (ARFs). The processor has two different voltage/frequency domains. The computation domain with 512 PEs operates at near-threshold voltage (NTV) (0.4 V) and 60-MHz frequency to increase energy efficiency, while the rest of the processors including 848-KB SRAMs run at 0.7 V and 120-MHz frequency to increase both on-chip and off-chip memory bandwidths. The measurement results show that our processor is capable of running AlexNet at 831 GOPS/W, VGG-16 at 1151 GOPS/W, ResNet-18 at 1004 GOPS/W, and MobileNet at 948 GOPS/W energy efficiency.
- DOI
- 10.1109/TVLSI.2019.2935251
- Appears in Collections:
- 인공지능대학 > 컴퓨터공학과 > Journal papers
- Files in This Item:
There are no files associated with this item.
- Export
- RIS (EndNote)
- XLS (Excel)
- XML