View : 818 Download: 292
Prediction of cognitive impairment via deep learning trained with multi-center neuropsychological test data
- Title
- Prediction of cognitive impairment via deep learning trained with multi-center neuropsychological test data
- Authors
- Kang, Min Ju; Kim, Sang Yun; Na, Duk L.; Kim, Byeong C.; Yang, Dong Won; Kim, Eun-Joo; Na, Hae Ri; Han, Hyun Jeong; Lee, Jae-Hong; Kim, Jong Hun; Park, Kee Hyung; Park, Kyung Won; Han, Seol-Heui; Kim, Seong Yoon; Yoon, Soo Jin; Yoon, Bora; Seo, Sang Won; Moon, So Young; Yang, YoungSoon; Shim, Yong S.; Baek, Min Jae; Jeong, Jee Hyang; Choi, Seong Hye; Youn, Young Chul
- Ewha Authors
- 정지향
- SCOPUS Author ID
- 정지향
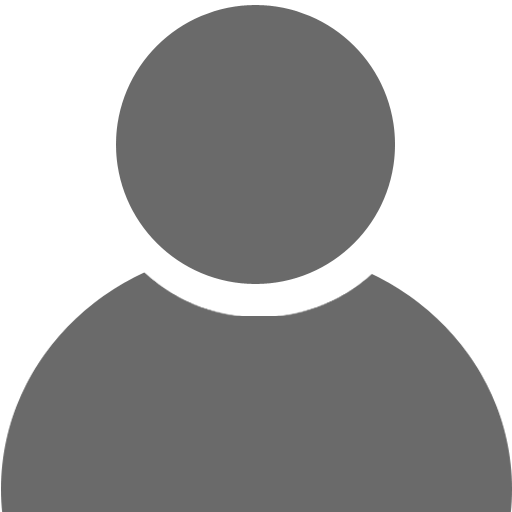
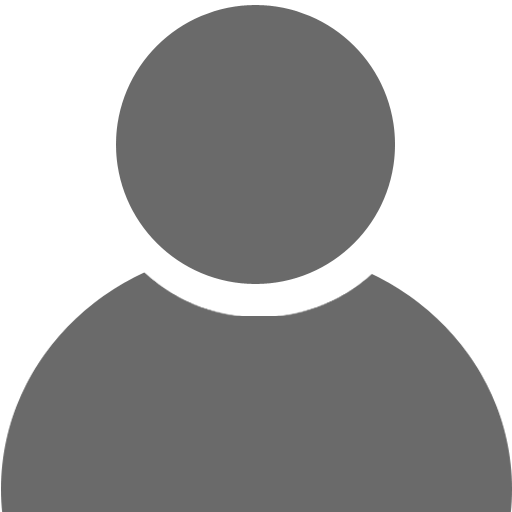
- Issue Date
- 2019
- Journal Title
- BMC MEDICAL INFORMATICS AND DECISION MAKING
- ISSN
- 1472-6947
- Citation
- BMC MEDICAL INFORMATICS AND DECISION MAKING vol. 19, no. 1
- Keywords
- Machine learning; Neuropsychological test; Dementia; Mild cognitive impairment; Alzheimer's disease
- Publisher
- BMC
- Indexed
- SCIE; SCOPUS

- Document Type
- Article
- Abstract
- Background Neuropsychological tests (NPTs) are important tools for informing diagnoses of cognitive impairment (CI). However, interpreting NPTs requires specialists and is thus time-consuming. To streamline the application of NPTs in clinical settings, we developed and evaluated the accuracy of a machine learning algorithm using multi-center NPT data. Methods Multi-center data were obtained from 14,926 formal neuropsychological assessments (Seoul Neuropsychological Screening Battery), which were classified into normal cognition (NC), mild cognitive impairment (MCI) and Alzheimer's disease dementia (ADD). We trained a machine learning model with artificial neural network algorithm using TensorFlow () to distinguish cognitive state with the 46-variable data and measured prediction accuracies from 10 randomly selected datasets. The features of the NPT were listed in order of their contribution to the outcome using Recursive Feature Elimination. Results The ten times mean accuracies of identifying CI (MCI and ADD) achieved by 96.66 +/- 0.52% of the balanced dataset and 97.23 +/- 0.32% of the clinic-based dataset, and the accuracies for predicting cognitive states (NC, MCI or ADD) were 95.49 +/- 0.53 and 96.34 +/- 1.03%. The sensitivity to the detection CI and MCI in the balanced dataset were 96.0 and 96.0%, and the specificity were 96.8 and 97.4%, respectively. The 'time orientation' and '3-word recall' score of MMSE were highly ranked features in predicting CI and cognitive state. The twelve features reduced from 46 variable of NPTs with age and education had contributed to more than 90% accuracy in predicting cognitive impairment. Conclusions The machine learning algorithm for NPTs has suggested potential use as a reference in differentiating cognitive impairment in the clinical setting.
- DOI
- 10.1186/s12911-019-0974-x
- Appears in Collections:
- 의과대학 > 의학과 > Journal papers
- Files in This Item:
-
Prediction of cognitive impairment.pdf(2.07 MB)
Download
- Export
- RIS (EndNote)
- XLS (Excel)
- XML