View : 568 Download: 190
MLACP: Machine-learning-based prediction of anticancer peptides
- Title
- MLACP: Machine-learning-based prediction of anticancer peptides
- Authors
- Manavalan B.; Basith S.; Shin T.H.; Choi S.; Kim M.O.; Lee G.
- Ewha Authors
- 최선
- SCOPUS Author ID
- 최선
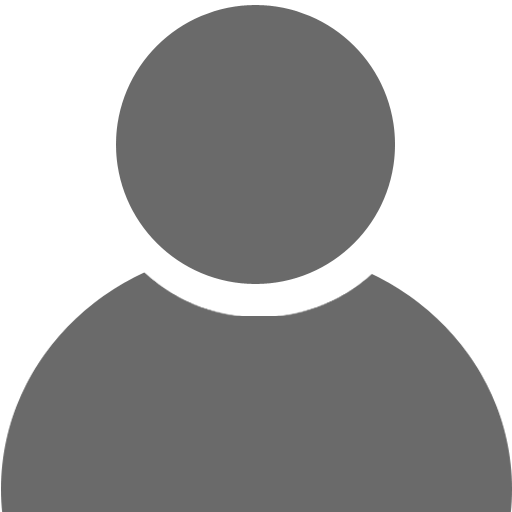
- Issue Date
- 2017
- Journal Title
- Oncotarget
- ISSN
- 1949-2553
- Citation
- Oncotarget vol. 8, no. 44, pp. 77121 - 77136
- Keywords
- Anticancer peptides; Hybrid model; Machine-learning parameters; Random forest; Support vector machine
- Publisher
- Impact Journals LLC
- Indexed
- SCIE; SCOPUS

- Document Type
- Article
- Abstract
- Cancer is the second leading cause of death globally, and use of therapeutic peptides to target and kill cancer cells has received considerable attention in recent years. Identification of anticancer peptides (ACPs) through wet-lab experimentation is expensive and often time consuming; therefore, development of an efficient computational method is essential to identify potential ACP candidates prior to in vitro experimentation. In this study, we developed support vector machine- and random forest-based machine-learning methods for the prediction of ACPs using the features calculated from the amino acid sequence, including amino acid composition, dipeptide composition, atomic composition, and physicochemical properties. We trained our methods using the Tyagi-B dataset and determined the machine parameters by 10-fold cross-validation. Furthermore, we evaluated the performance of our methods on two benchmarking datasets, with our results showing that the random forest-based method outperformed the existing methods with an average accuracy and Matthews correlation coefficient value of 88.7% and 0.78, respectively. To assist the scientific community, we also developed a publicly accessible web server at www.thegleelab. org/MLACP.html. © Manavalan et al.
- DOI
- 10.18632/oncotarget.20365
- Appears in Collections:
- 약학대학 > 약학과 > Journal papers
- Files in This Item:
-
MLACP.pdf(4.32 MB)
Download
- Export
- RIS (EndNote)
- XLS (Excel)
- XML