View : 1 Download: 0
Machine learning model for osteoporosis diagnosis based on bone turnover markers
- Title
- Machine learning model for osteoporosis diagnosis based on bone turnover markers
- Authors
- Baik; Seung Min; Kwon; Hi Jeong; Kim; Yeongsic; Lee; Jehoon; Park; Young Hoon; Dong Jin
- Ewha Authors
- 박영훈; 백승민
- SCOPUS Author ID
- 박영훈
; 백승민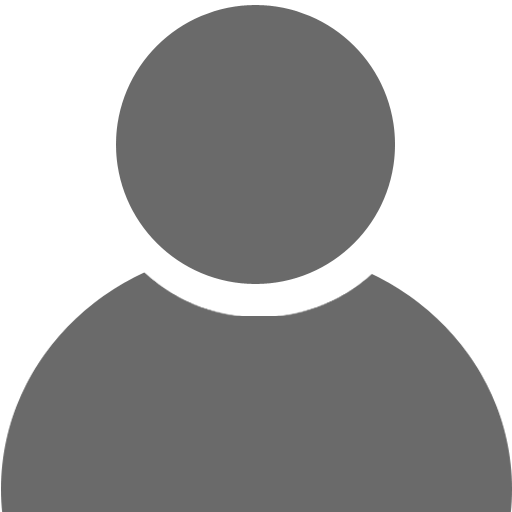
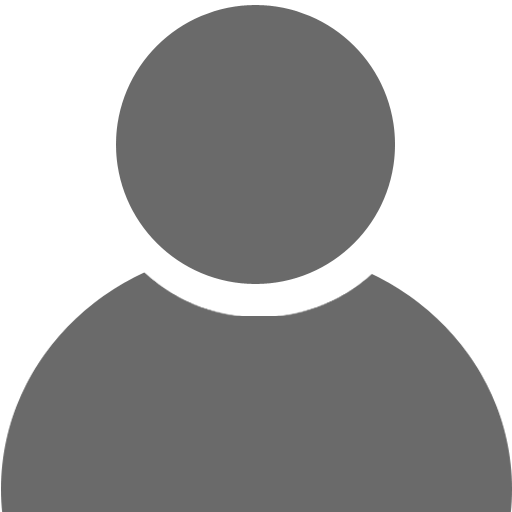
- Issue Date
- 2024
- Journal Title
- Health Informatics Journal
- ISSN
- 1460-4582
- Citation
- Health Informatics Journal vol. 30, no. 3
- Keywords
- artificial intelligence; bone turnover marker; ensemble technique; machine learning; osteoporosis diagnosis
- Indexed
- SCIE; SCOPUS

- Document Type
- Article
- Abstract
- To assess the diagnostic utility of bone turnover markers (BTMs) and demographic variables for identifying individuals with osteoporosis. A cross-sectional study involving 280 participants was conducted. Serum BTM values were obtained from 88 patients with osteoporosis and 192 controls without osteoporosis. Six machine learning models, including extreme gradient boosting (XGBoost), light gradient boosting machine (LGBM), CatBoost, random forest, support vector machine, and k-nearest neighbors, were employed to evaluate osteoporosis diagnosis. The performance measures included the area under the receiver operating characteristic curve (AUROC), F1-score, and accuracy. After AUROC optimization, LGBM exhibited the highest AUROC of 0.706. Post F1-score optimization, LGBM’s F1-score was improved from 0.50 to 0.65. Combining the top three optimized models (LGBM, XGBoost, and CatBoost) resulted in an AUROC of 0.706, an F1-score of 0.65, and an accuracy of 0.73. BTMs, along with age and sex, were found to contribute significantly to osteoporosis diagnosis. This study demonstrates the potential of machine learning models utilizing BTMs and demographic variables for diagnosing preexisting osteoporosis. The findings highlight the clinical relevance of accessible clinical data in osteoporosis assessment, providing a promising tool for early diagnosis and management. © The Author(s) 2024.
- DOI
- 10.1177/14604582241270778
- Appears in Collections:
- 의료원 > 의료원 > Journal papers
- Files in This Item:
There are no files associated with this item.
- Export
- RIS (EndNote)
- XLS (Excel)
- XML