View : 91 Download: 0
Enhancing Imbalanced Sentiment Analysis: A GPT-3-Based Sentence-by-Sentence Generation Approach
- Title
- Enhancing Imbalanced Sentiment Analysis: A GPT-3-Based Sentence-by-Sentence Generation Approach
- Authors
- Suhaeni, Cici; Yong, Hwan-Seung
- Ewha Authors
- 용환승
- SCOPUS Author ID
- 용환승
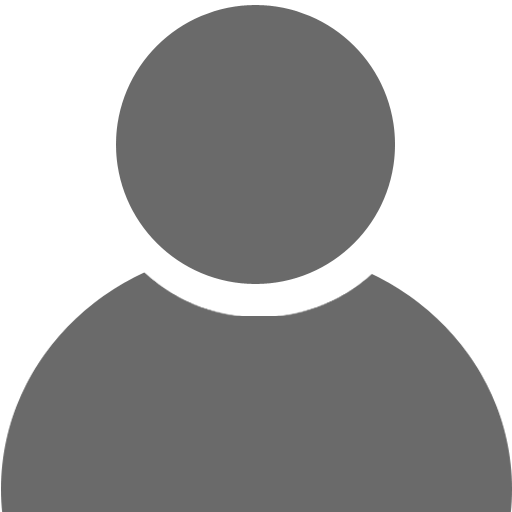
- Issue Date
- 2024
- Journal Title
- APPLIED SCIENCES-BASEL
- ISSN
- 2076-3417
- Citation
- APPLIED SCIENCES-BASEL vol. 14, no. 2
- Keywords
- GPT-3; imbalanced sentiment analysis; sentiment analysis; synthetic data generation; text classification; text generation; large language model (LLM)
- Publisher
- MDPI
- Indexed
- SCIE; SCOPUS

- Document Type
- Article
- Abstract
- This study addresses the challenge of class imbalance in sentiment analysis by utilizing synthetic data to balance training datasets. We introduce an innovative approach using the GPT-3 model's sentence-by-sentence generation technique to generate synthetic data, specifically targeting underrepresented negative and neutral sentiments. Our method aims to align these minority classes with the predominantly positive sentiment class in a Coursera course review dataset, with the goal of enhancing the performance of sentiment classification. This research demonstrates that our proposed method successfully enhances sentiment classification performance, as evidenced by improved accuracy and F1-score metrics across five deep-learning models. However, when compared to our previous research utilizing fine-tuning techniques, the current method shows a relative shortfall. The fine-tuning approach yields better results in all models tested, indicating the importance of data novelty and diversity in synthetic data generation. In terms of the deep-learning model used for classification, the notable finding is the significant performance improvement of the Recurrent Neural Network (RNN) model compared to other models like CNN, LSTM, BiLSTM, and GRU, highlighting the impact of the model choice and architecture depth. This study emphasizes the critical role of synthetic data quality and strategic deep-learning model implementation in sentiment analysis. The results suggest that the careful consideration of training data and model attributes is vital for optimal sentiment classification.
- DOI
- 10.3390/app14020622|http://dx.doi.org/10.3390/app14020622
- Appears in Collections:
- 인공지능대학 > 컴퓨터공학과 > Journal papers
- Files in This Item:
There are no files associated with this item.
- Export
- RIS (EndNote)
- XLS (Excel)
- XML