View : 250 Download: 0
Data-driven machine learning models for the prediction of hydrogen solubility in aqueous systems of varying salinity: Implications for underground hydrogen storage
- Title
- Data-driven machine learning models for the prediction of hydrogen solubility in aqueous systems of varying salinity: Implications for underground hydrogen storage
- Authors
- Thanh, Hung Vo; Zhang, Hemeng; Dai, Zhenxue; Zhang, Tao; Tangparitkul, Suparit; Min, Baehyun
- Ewha Authors
- 민배현
- SCOPUS Author ID
- 민배현
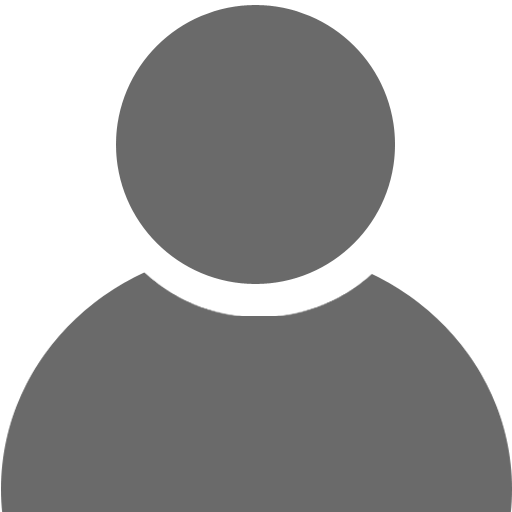
- Issue Date
- 2024
- Journal Title
- INTERNATIONAL JOURNAL OF HYDROGEN ENERGY
- ISSN
- 0360-3199
1879-3487
- Citation
- INTERNATIONAL JOURNAL OF HYDROGEN ENERGY vol. 55, pp. 1422 - 1433
- Keywords
- Underground hydrogen storage; Machine learning; Hydrogen solubility; Adaptive gradient boosting; Random forest
- Publisher
- PERGAMON-ELSEVIER SCIENCE LTD
- Indexed
- SCIE; SCOPUS

- Document Type
- Article
- Abstract
- Hydrogen is a clean and sustainable renewable energy source with significant potential for use in energy storage applications because of its high energy density. In particular, underground hydrogen storage via the dissolution of hydrogen gas in an aqueous solution has been identified as a promising strategy to address the difficulties associated with large-scale energy storage. However, this process requires the accurate prediction of the solubility of hydrogen in aqueous solutions, which is affected by a range of factors, including temperature, pressure, and the presence of solutes. The present study thus aimed to effectively predict the solubility of hydrogen in aqueous solutions that vary in their salinity by employing a machine learning approach. Four machine learning models were developed and tested: adaptive gradient boosting (Adaboost), gradient boosting, random forest, and extreme gradient boosting. The performance of each model was quantified in terms of their coefficient of determination (R2), root mean square error (RMSE), and mean absolute error (MAE). The Adaboost algorithm exhibited superior performance across all metrics, with an R2 of 0.994, MAE of 0.006, and RMSE of 0.018. A Williams plot detected only 18 outliers in the Adaboost predictions from a total of 255 data points. These results indicate that machine learning techniques have the potential to serve as a valuable tool in the prediction of hydrogen solubility in aqueous solutions for underground hydrogen storage, facilitating the development of smart, cost-effective, and safe hydrogen storage technologies.
- DOI
- 10.1016/j.ijhydene.2023.12.131|http://dx.doi.org/10.1016/j.ijhydene.2023.12.131
- Appears in Collections:
- 공과대학 > 기후에너지시스템공학과 > Journal papers
- Files in This Item:
There are no files associated with this item.
- Export
- RIS (EndNote)
- XLS (Excel)
- XML