View : 121 Download: 0
Orthognathic surgical planning using graph CNN with dual embedding module: External validations with multi-hospital datasets
- Title
- Orthognathic surgical planning using graph CNN with dual embedding module: External validations with multi-hospital datasets
- Authors
- Kim; In-Hwan; Jun-Sik; Jeong; Jiheon; Park; Jae-Woo; Kanggil; Cho; Jin-Hyoung; Hong; Mihee; Kang; Kyung-Hwa; Minji; Su-Jung; Yoon-Ji; Sung; Sang-Jin; Young Ho; Lim; Sung-Hoon; Baek; Seung-Hak; Namkug
- Ewha Authors
- 김민지
- SCOPUS Author ID
- 김민지
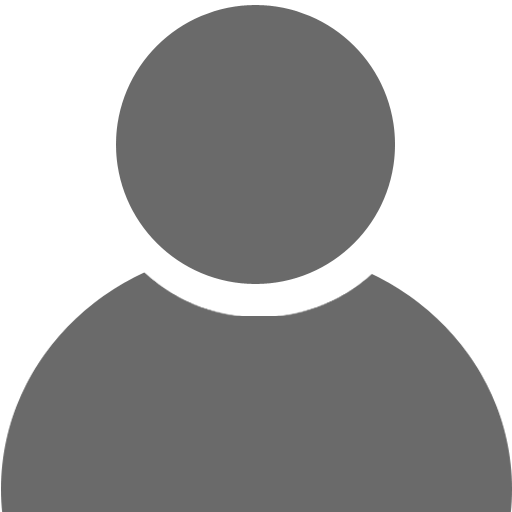
- Issue Date
- 2023
- Journal Title
- Computer Methods and Programs in Biomedicine
- ISSN
- 0169-2607
- Citation
- Computer Methods and Programs in Biomedicine vol. 242
- Keywords
- Cephalometry; Deep learning; Dual embedding module; Graph convolution neural network; Maxillofacial surgery; Multicenter study; Orthognathic surgery; Surgical prediction
- Publisher
- Elsevier Ireland Ltd
- Indexed
- SCIE; SCOPUS

- Document Type
- Article
- Abstract
- Background and objective: Despite recent development of AI, prediction of the surgical movement in the maxilla and mandible by OGS might be more difficult than that of tooth movement by orthodontic treatment. To evaluate the prediction accuracy of the surgical movement using pairs of pre-(T0) and post-surgical (T1) lateral cephalograms (lat-ceph) of orthognathic surgery (OGS) patients and dual embedding module-graph convolution neural network (DEM-GCNN) model. Methods: 599 pairs from 3 institutions were used as training, internal validation, and internal test sets and 201 pairs from other 6 institutions were used as external test set. DEM-GCNN model (IEM, learning the lat-ceph images; LTEM, learning the landmarks) was developed to predict the amount and direction of surgical movement of ANS and PNS in the maxilla and B-point and Md1crown in the mandible. The distance between T1 landmark coordinates actually moved by OGS (ground truth) and predicted by DEM-GCNN model and pre-existed CNN-based Model-C (learning the lat-ceph images) was compared. Results: In both internal and external tests, DEM-GCNN did not exhibit significant difference from ground truth in all landmarks (ANS, PNS, B-point, Md1crown, all P > 0.05). When the accumulated successful detection rate for each landmark was compared, DEM-GCNN showed higher values than Model-C in both the internal and external tests. In violin plots exhibiting the error distribution of the prediction results, both internal and external tests showed that DEM-GCNN had significant performance improvement in PNS, ANS, B-point, Md1crown than Model-C. DEM-GCNN showed significantly lower prediction error values than Model-C (one-jaw surgery, B-point, Md1crown, all P < 0.005; two-jaw surgery, PNS, ANS, all P < 0.05; B point, Md1crown, all P < 0.005). Conclusion: We developed a robust OGS planning model with maximized generalizability despite diverse qualities of lat-cephs from 9 institutions. © 2023 Elsevier B.V.
- DOI
- 10.1016/j.cmpb.2023.107853
- Appears in Collections:
- 의과대학 > 의학과 > Journal papers
- Files in This Item:
There are no files associated with this item.
- Export
- RIS (EndNote)
- XLS (Excel)
- XML