View : 186 Download: 0
Deep learning of sleep apnea-hypopnea events for accurate classification of obstructive sleep apnea and determination of clinical severity
- Title
- Deep learning of sleep apnea-hypopnea events for accurate classification of obstructive sleep apnea and determination of clinical severity
- Authors
- Yook; Soonhyun; Kim; Dongyeop; Gupte; Chaitanya; Joo; Eun Yeon; Hosung
- Ewha Authors
- 김동엽
- SCOPUS Author ID
- 김동엽
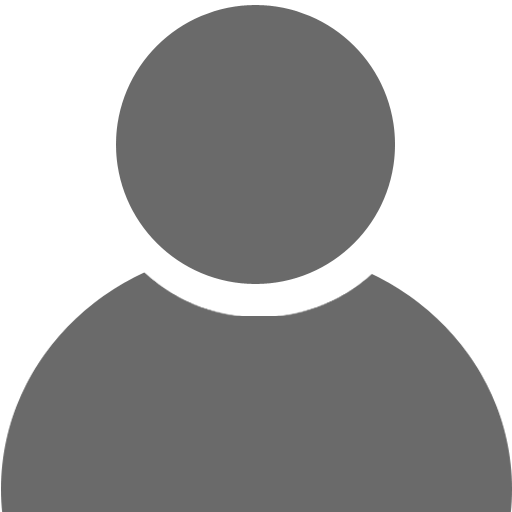
- Issue Date
- 2024
- Journal Title
- Sleep Medicine
- ISSN
- 1389-9457
- Citation
- Sleep Medicine vol. 114, pp. 211 - 219
- Keywords
- Apnea-hypopnea index; Biosignals; Deep learning; Machine learning; Obstructive sleep apnea; Scalogram
- Publisher
- Elsevier B.V.
- Indexed
- SCIE; SCOPUS

- Document Type
- Article
- Abstract
- Background: /Objective: Automatic apnea/hypopnea events classification, crucial for clinical applications, often faces challenges, particularly in hypopnea detection. This study aimed to evaluate the efficiency of a combined approach using nasal respiration flow (RF), peripheral oxygen saturation (SpO2), and ECG signals during polysomnography (PSG) for improved sleep apnea/hypopnea detection and obstructive sleep apnea (OSA) severity screening. Methods: An Xception network was trained using main features from RF, SpO2, and ECG signals obtained during PSG. In addition, we incorporated demographic data for enhanced performance. The detection of apnea/hypopnea events was based on RF and SpO2 feature sets, while the screening and severity categorization of OSA utilized predicted apnea/hypopnea events in conjunction with demographic data. Results: Using RF and SpO2 feature sets, our model achieved an accuracy of 94 % in detecting apnea/hypopnea events. For OSA screening, an exceptional accuracy of 99 % and an AUC of 0.99 were achieved. OSA severity categorization yielded an accuracy of 93 % and an AUC of 0.91, with no misclassification between normal and mild OSA versus moderate and severe OSA. However, classification errors predominantly arose in cases with hypopnea-prevalent participants. Conclusions: The proposed method offers a robust automatic detection system for apnea/hypopnea events, requiring fewer sensors than traditional PSG, and demonstrates exceptional performance. Additionally, the classification algorithms for OSA screening and severity categorization exhibit significant discriminatory capacity. © 2024 Elsevier B.V.
- DOI
- 10.1016/j.sleep.2024.01.015
- Appears in Collections:
- 의료원 > 의료원 > Journal papers
- Files in This Item:
There are no files associated with this item.
- Export
- RIS (EndNote)
- XLS (Excel)
- XML