View : 165 Download: 0
Explainable Artificial Intelligence (XAI) Surrogate Models for Chemical Process Design and Analysis; [화학 공정 설계 및 분석을 위한 설명 가능한 인공지능 대안 모델]
- Title
- Explainable Artificial Intelligence (XAI) Surrogate Models for Chemical Process Design and Analysis; [화학 공정 설계 및 분석을 위한 설명 가능한 인공지능 대안 모델]
- Authors
- Ko; Yuna; Na; Jonggeol
- Ewha Authors
- 나종걸
- SCOPUS Author ID
- 나종걸
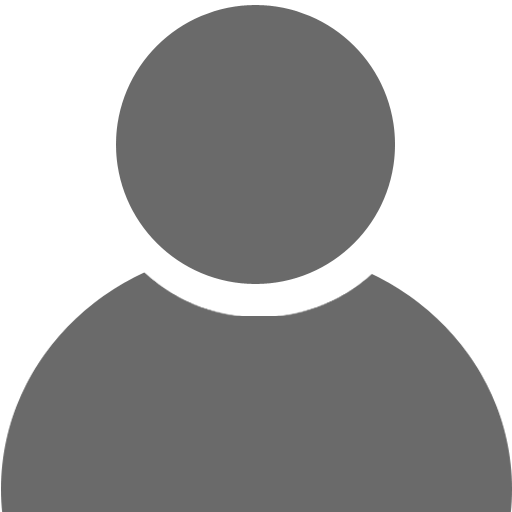
- Issue Date
- 2023
- Journal Title
- Korean Chemical Engineering Research
- ISSN
- 0304-128X
- Citation
- Korean Chemical Engineering Research vol. 61, no. 4, pp. 542 - 549
- Keywords
- Process analysis; Process systems engineering; Surrogate modeling; XAI
- Publisher
- Korean Institute of Chemical Engineers
- Indexed
- SCOPUS; KCI

- Document Type
- Article
- Abstract
- Since the growing interest in surrogate modeling, there has been continuous research aimed at simulating nonlinear chemical processes using data-driven machine learning. However, the opaque nature of machine learning models, which limits their interpretability, poses a challenge for their practical application in industry. Therefore, this study aims to analyze chemical processes using Explainable Artificial Intelligence (XAI), a concept that improves interpretability while ensuring model accuracy. While conventional sensitivity analysis of chemical processes has been limited to calculating and ranking the sensitivity indices of variables, we propose a methodology that utilizes XAI to not only perform global and local sensitivity analysis, but also examine the interactions among variables to gain physical insights from the data. For the ammonia synthesis process, which is the target process of the case study, we set the temperature of the preheater leading to the first reactor and the split ratio of the cold shot to the three reactors as process variables. By integrating Matlab and Aspen Plus, we obtained data on ammonia production and the maximum temperatures of the three reactors while systematically varying the process variables. We then trained tree-based models and performed sensitivity analysis using the SHAP technique, one of the XAI methods, on the most accurate model. The global sensitivity analysis showed that the preheater temperature had the greatest effect, and the local sensitivity analysis provided insights for defining the ranges of process variables to improve productivity and prevent overheating. By constructing alternative models for chemical processes and using XAI for sensitivity analysis, this work contributes to providing both quantitative and qualitative feedback for process optimization. © 2023 Korean Institute of Chemical Engineers. All rights reserved.
- DOI
- 10.9713/kcer.2023.61.4.542
- Appears in Collections:
- 공과대학 > 화공신소재공학과 > Journal papers
- Files in This Item:
There are no files associated with this item.
- Export
- RIS (EndNote)
- XLS (Excel)
- XML