View : 316 Download: 0
Automated cryptocurrency trading approach using ensemble deep reinforcement learning: Learn to understand candlesticks
- Title
- Automated cryptocurrency trading approach using ensemble deep reinforcement learning: Learn to understand candlesticks
- Authors
- Jing, Liu; Kang, Yuncheol
- Ewha Authors
- 강윤철
- SCOPUS Author ID
- 강윤철
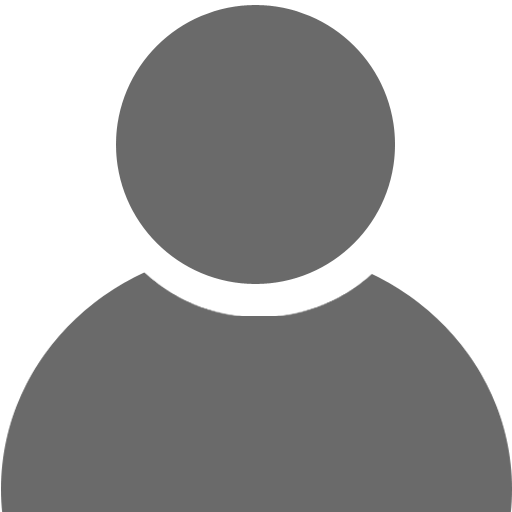
- Issue Date
- 2024
- Journal Title
- EXPERT SYSTEMS WITH APPLICATIONS
- ISSN
- 0957-4174
1873-6793
- Citation
- EXPERT SYSTEMS WITH APPLICATIONS vol. 237
- Keywords
- Automated trading; Candlestick images; Cryptocurrency; Deep reinforcement learning; Ensemble approach
- Publisher
- PERGAMON-ELSEVIER SCIENCE LTD
- Indexed
- SCIE; SCOPUS

- Document Type
- Article
- Abstract
- Despite their high risk, cryptocurrencies have gained popularity as viable trading options. Cryptocurrencies are digital assets that experience significant fluctuations in a market operating 24 h a day. Recently, considerable attention has been paid to developing trading bots using machine-learning-based artificial intelligence. Previous studies have employed machine learning techniques to predict financial market trends or make trading decisions, primarily using numerical data extracted from candlesticks. However, these data often overlook the temporal and spatial information of candlesticks, leading to a limited understanding of their significance. In this study, we utilize multi-resolution candlestick images containing temporal and spatial information. Our rationale for using visual information from candlestick charts is to replicate the decision-making processes of human trading experts. To achieve this, we employ deep reinforcement learning algorithms to generate trading signals based on a state vector that includes embedded candlestick-chart images. The trading signal is generated using a multi-agent weighted voting ensemble approach. We test the proposed approach on two BTC/USDT datasets under both bullish and bearish market scenarios. Additionally, we use an attention-based technique to identify significant areas in the candlestick images targeted by the proposed approach. Our findings demonstrate that models using candlestick images 'as-is', outperform those using raw numeric data and other baseline models.
- DOI
- 10.1016/j.eswa.2023.121373
- Appears in Collections:
- 경영대학 > 경영학전공 > Journal papers
- Files in This Item:
There are no files associated with this item.
- Export
- RIS (EndNote)
- XLS (Excel)
- XML