View : 323 Download: 0
Age-integrated artificial intelligence framework for sleep stage classification and obstructive sleep apnea screening
- Title
- Age-integrated artificial intelligence framework for sleep stage classification and obstructive sleep apnea screening
- Authors
- Kang, Chaewon; An, Sora; Kim, Hyeon Jin; Devi, Maithreyee; Cho, Aram; Hwang, Sungeun; Lee, Hyang Woon
- Ewha Authors
- 이향운; 안소라; 황성은
- SCOPUS Author ID
- 이향운
; 안소라
; 황성은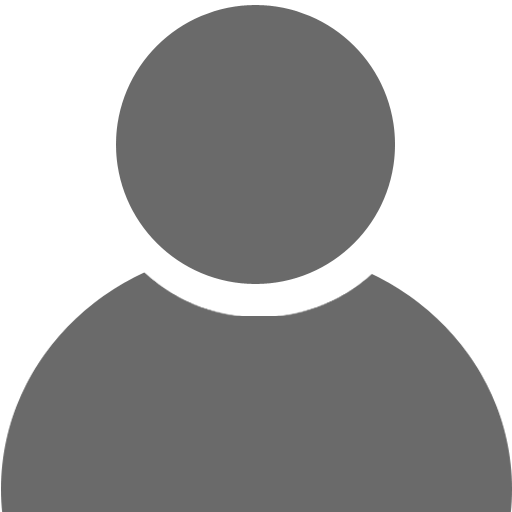
- Issue Date
- 2023
- Journal Title
- FRONTIERS IN NEUROSCIENCE
- ISSN
- 1662-453X
- Citation
- FRONTIERS IN NEUROSCIENCE vol. 17
- Keywords
- polysomnography; electroencephalography; sleep staging; obstructive sleep apnea; age-integrated; machine learning; artificial intelligence
- Publisher
- FRONTIERS MEDIA SA
- Indexed
- SCIE; SCOPUS

- Document Type
- Article
- Abstract
- IntroductionSleep is an essential function to sustain a healthy life, and sleep dysfunction can cause various physical and mental issues. In particular, obstructive sleep apnea (OSA) is one of the most common sleep disorders and, if not treated in a timely manner, OSA can lead to critical problems such as hypertension or heart disease. MethodsThe first crucial step in evaluating individuals' quality of sleep and diagnosing sleep disorders is to classify sleep stages using polysomnographic (PSG) data including electroencephalography (EEG). To date, such sleep stage scoring has been mainly performed manually via visual inspection by experts, which is not only a time-consuming and laborious process but also may yield subjective results. Therefore, we have developed a computational framework that enables automatic sleep stage classification utilizing the power spectral density (PSD) features of sleep EEG based on three different learning algorithms: support vector machine, k-nearest neighbors, and multilayer perceptron (MLP). In particular, we propose an integrated artificial intelligence (AI) framework to further inform the risk of OSA based on the characteristics in automatically scored sleep stages. Given the previous finding that the characteristics of sleep EEG differ by age group, we employed a strategy of training age-specific models (younger and older groups) and a general model and comparing their performance. ResultsThe performance of the younger age-specific group model was similar to that of the general model (and even higher than the general model at certain stages), but the performance of the older age-specific group model was rather low, suggesting that bias in individual variables, such as age bias, should be considered during model training. Our integrated model yielded an accuracy of 73% in sleep stage classification and 73% in OSA screening when MLP algorithm was applied, which indicates that patients with OSA could be screened with the corresponding accuracy level only with sleep EEG without respiration-related measures. DiscussionThe current outcomes demonstrate the feasibility of AI-based computational studies that when combined with advances in wearable devices and relevant technologies could contribute to personalized medicine by not only assessing an individuals' sleep status conveniently at home but also by alerting them to the risk of sleep disorders and enabling early intervention.
- DOI
- 10.3389/fnins.2023.1059186|http://dx.doi.org/10.3389/fnins.2023.1059186
- Appears in Collections:
- 의과대학 > 의학과 > Journal papers
- Files in This Item:
There are no files associated with this item.
- Export
- RIS (EndNote)
- XLS (Excel)
- XML