View : 303 Download: 0
Machine-learning-based prediction of oil recovery factor for experimental CO2-Foam chemical EOR: Implications for carbon utilization projects
- Title
- Machine-learning-based prediction of oil recovery factor for experimental CO2-Foam chemical EOR: Implications for carbon utilization projects
- Authors
- Vo Thanh H.; Sheini Dashtgoli D.; Zhang H.; Min B.
- Ewha Authors
- 민배현
- SCOPUS Author ID
- 민배현
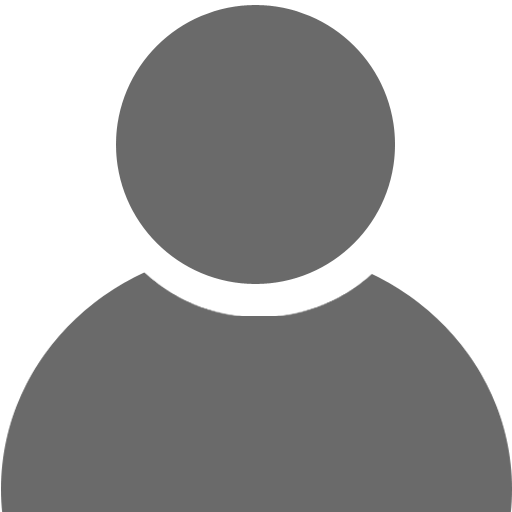
- Issue Date
- 2023
- Journal Title
- Energy
- ISSN
- 3605-5442
- Citation
- Energy vol. 278
- Keywords
- CFNN-BR; CFNN-LM; CO<sub>2</sub>-EOR; CO<sub>2</sub>-Foam experiments; GRNN; XGBoost
- Publisher
- Elsevier Ltd
- Indexed
- SCIE; SCOPUS

- Document Type
- Article
- Abstract
- Enhanced oil recovery (EOR) using CO2 injection is promising with economic and environmental benefits as an active climate-change mitigation approach. Nevertheless, the low sweep efficiency of CO2 injection remains a challenge. CO2-foam injection has been proposed as a remedy, but its laboratory screening for specific reservoirs is costly and time-consuming. In this study, machine-learning models are employed to predict oil recovery factor (ORF) during CO2-foam flooding cost-effectively and accurately. Four models, including general regression neural network (GRNN), cascade forward neural network with Levenberg–Marquardt optimization (CFNN-LM), cascade forward neural network with Bayesian regularization (CFNN-BR), and extreme gradient boosting (XGBoost), are evaluated based on experimental data from previous studies. Results demonstrate that the GRNN model outperforms the others, with an overall mean absolute error of 0.059 and an R2 of 0.9999. The GRNN model's applicability domain is verified using a Williams plot, and an uncertainty analysis for CO2-foam flooding projects is conducted. The novelty of this study lies in developing a machine-learning-based approach that provides an accurate and cost-effective prediction of ORF in CO2-foam experiments. This approach has the potential to significantly reduce screening costs and time required for CO2-foam injection, making it a more viable carbon utilization and EOR strategy. © 2023 Elsevier Ltd
- DOI
- 10.1016/j.energy.2023.127860
- Appears in Collections:
- 공과대학 > 기후에너지시스템공학과 > Journal papers
- Files in This Item:
There are no files associated with this item.
- Export
- RIS (EndNote)
- XLS (Excel)
- XML