View : 390 Download: 0
Learning disentangled skills for hierarchical reinforcement learning through trajectory autoencoder with weak labels
- Title
- Learning disentangled skills for hierarchical reinforcement learning through trajectory autoencoder with weak labels
- Authors
- Song; Wonil; Jeon; Sangryul; Choi; Hyesong; Sohn; Kwanghoon; Min; Dongbo
- Ewha Authors
- 민동보
- SCOPUS Author ID
- 민동보
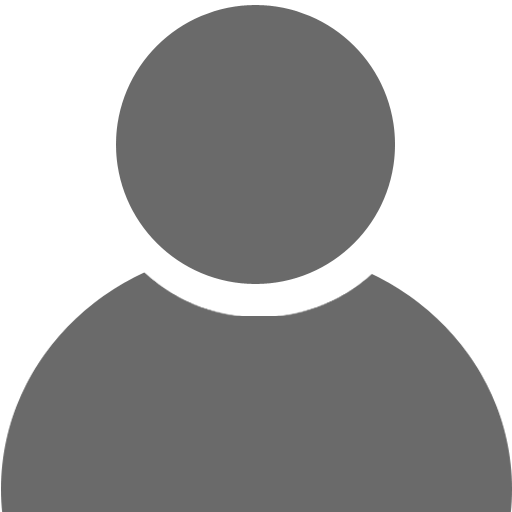
- Issue Date
- 2023
- Journal Title
- Expert Systems with Applications
- ISSN
- 0957-4174
- Citation
- Expert Systems with Applications vol. 230
- Keywords
- Deep reinforcement learning; Disentangled representation; Hierarchical reinforcement learning; Planning; Skill learning; Variational autoencoder; Weak label
- Publisher
- Elsevier Ltd
- Indexed
- SCIE; SCOPUS

- Document Type
- Article
- Abstract
- Typically, hierarchical reinforcement learning (RL) requires skills that are applicable to various downstream tasks. Although several recent studies have proposed the supervised and unsupervised learning of such skills, the learned skills are often entangled, which hinders their interpretation. To alleviate this, we propose a novel method to use weak labels for learning disentangled skills from the continuous latent representations of trajectories. To this end, we extended a trajectory variational autoencoder (VAE) to impose an inductive bias using weak labels, which explicitly enforces the disentangling of the trajectory representations into factors of interest intended for the model to learn. Using the latent representations as skills, a skill-based policy network is trained to generate trajectories similar to the learned decoder of the trajectory VAE. Furthermore, using the disentangled skill, we propose a skill repetition that can expand the entire trajectories generated by the policy at test time, resulting in an effective planning strategy. Experiments were performed on several challenging navigation tasks in mazes, and the results demonstrate the effectiveness of our method at solving hierarchical RL problems even with a long horizon and sparse rewards. © 2023 Elsevier Ltd
- DOI
- 10.1016/j.eswa.2023.120625
- Appears in Collections:
- 인공지능대학 > 컴퓨터공학과 > Journal papers
- Files in This Item:
There are no files associated with this item.
- Export
- RIS (EndNote)
- XLS (Excel)
- XML