View : 447 Download: 0
MM-Net: Multiframe and Multimask-Based Unsupervised Deep Denoising for Low-Dose Computed Tomography
- Title
- MM-Net: Multiframe and Multimask-Based Unsupervised Deep Denoising for Low-Dose Computed Tomography
- Authors
- Jeon S.-Y.; Kim W.; Choi J.-H.
- Ewha Authors
- 최장환
- SCOPUS Author ID
- 최장환
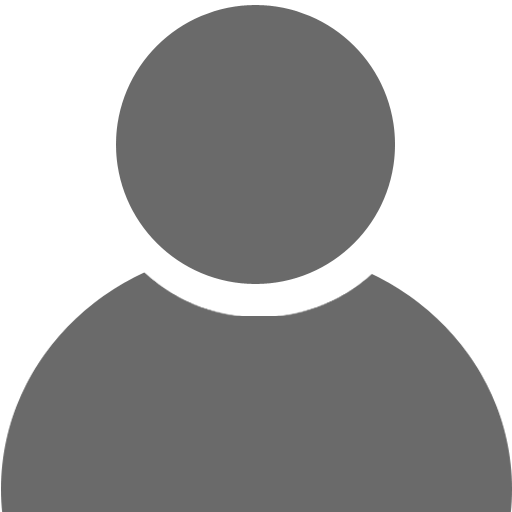
- Issue Date
- 2023
- Journal Title
- IEEE Transactions on Radiation and Plasma Medical Sciences
- ISSN
- 2469-7311
- Citation
- IEEE Transactions on Radiation and Plasma Medical Sciences vol. 7, no. 3, pp. 296 - 306
- Keywords
- Artifact removal; deep learning (DL); image denoising; low-dose computed tomography (LDCT); unsupervised learning
- Publisher
- Institute of Electrical and Electronics Engineers Inc.
- Indexed
- SCOPUS

- Document Type
- Article
- Abstract
- Low-dose computed tomography (LDCT) is crucial due to the risk of radiation exposure to patients. However, the high noise level in LDCT images may reduce the image quality, leading to a less accurate diagnosis. Deep learning technology, especially supervised methods, has recently been widely accepted as a powerful tool for LDCT image denoising tasks. However, supervised methods require numerous paired datasets of LDCT and high-quality pristine CT images, which are rarely available in real-world clinical scenarios. This study presents an unsupervised learning-based framework, called MM-Net, consisting of two training steps for a volumetric LDCT denoising task. In the two-step training approach, we first train the initial denoising network multiscale attention U-Net (MSAU-Net) in a self-supervised manner to predict the noise-suppressed center slice with a neighboring multislice input. The second training step aims to train the U-Net-based final denoiser based on the pretrained MSAU-Net to improve the image quality by introducing new multipatch and multimask matching loss. Qualitative visual inspection and quantitative measures across texturally different domains of clinical and animal data reveal that the proposed MM-Net outperformed all competing state-of-the-art unsupervised algorithms. The unsupervised method also achieved denoising performance comparable to the representative supervised methods trained with ground-truth images. © 2017 IEEE.
- DOI
- 10.1109/TRPMS.2022.3224553
- Appears in Collections:
- 인공지능대학 > 인공지능학과 > Journal papers
- Files in This Item:
There are no files associated with this item.
- Export
- RIS (EndNote)
- XLS (Excel)
- XML