View : 285 Download: 113
A Short-Term Forecasting of Wind Power Outputs Based on Gradient Boosting Regression Tree Algorithms
- Title
- A Short-Term Forecasting of Wind Power Outputs Based on Gradient Boosting Regression Tree Algorithms
- Authors
- Park, Soyoung; Jung, Solyoung; Lee, Jaegul; Hur, Jin
- Ewha Authors
- 허진
- SCOPUS Author ID
- 허진
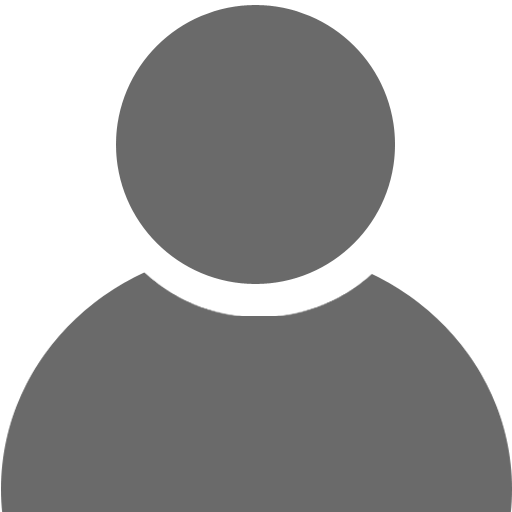
- Issue Date
- 2023
- Journal Title
- ENERGIES
- ISSN
- 1996-1073
- Citation
- ENERGIES vol. 16, no. 3
- Keywords
- renewable energy; wind-power forecasting; machine learning; gradient-boosting machine (GBM)
- Publisher
- MDPI
- Indexed
- SCIE; SCOPUS

- Document Type
- Article
- Abstract
- With growing interest in sustainability and net-zero emissions, there has been a global trend to integrate wind power into energy grids. However, challenges such as the intermittency of wind energy remain, which leads to a significant need for accurate wind-power forecasting. Therefore, this study focuses on creating a wind-power generation-forecasting model using a machine-learning algorithm. In this study, we used the gradient-boosting machine (GBM) algorithm to build a wind-power forecasting model. Time-series data with a 15 min interval from Jeju's wind farms were applied to the model as input data. The short-term forecasting model trained by the same month with the test set turns out to have the best performance, with an NMAE value of 5.15%. Furthermore, the forecasting results were applied to Jeju's power system to carry out a grid-security analysis. The improved accuracy of wind-power forecasting and its impact on the security of electrical grids in this study potentially contributes to greater integration of wind energy.
- DOI
- 10.3390/en16031132
- Appears in Collections:
- 공과대학 > 기후에너지시스템공학과 > Journal papers
- Files in This Item:
-
energies-16-01132-v3.pdf(2.21 MB)
Download
- Export
- RIS (EndNote)
- XLS (Excel)
- XML