View : 216 Download: 0
Explainable deep learning-based clinical decision support engine for MRI-base d automate d diagnosis of temporomandibular joint anterior disk displacement
- Title
- Explainable deep learning-based clinical decision support engine for MRI-base d automate d diagnosis of temporomandibular joint anterior disk displacement
- Authors
- Yoon, Kyubaek; Kim, Jae-Young; Kim, Sun-Jong; Huh, Jong-Ki; Kim, Jin-Woo; Choi, Jongeun
- Ewha Authors
- 김진우
- SCOPUS Author ID
- 김진우
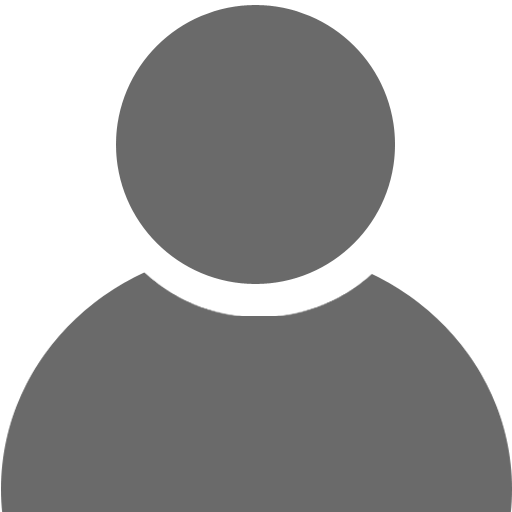
- Issue Date
- 2023
- Journal Title
- COMPUTER METHODS AND PROGRAMS IN BIOMEDICINE
- ISSN
- 0169-2607
1872-7565
- Citation
- COMPUTER METHODS AND PROGRAMS IN BIOMEDICINE vol. 233
- Keywords
- Temporomadibular joint; Anterior disc displacement; Clinical decision support system; Deep learning; Diagnosis; Explainable artificial intelligence
- Publisher
- ELSEVIER IRELAND LTD
- Indexed
- SCIE; SCOPUS

- Document Type
- Article
- Abstract
- Background and Objective: MRI is considered the gold standard for diagnosing anterior disc displacement (ADD), the most common temporomandibular joint (TMJ) disorder. However, even highly trained clinicians find it difficult to integrate the dynamic nature of MRI with the complicated anatomical features of the TMJ. As the first validated study for MRI-based automatic TMJ ADD diagnosis, we propose a clinical decision support engine that diagnoses TMJ ADD using MR images and provides heat maps as the visualized rationale of diagnostic predictions using explainable artificial intelligence. Methods: The engine builds on two deep learning models. The first deep learning model detects a region of interest (ROI) containing three TMJ components (i.e., temporal bone, disc, and condyle) in the entire sagittal MR image. The second deep learning model classifies TMJ ADD into three classes (i.e., normal, ADD without reduction, and ADD with reduction) within the detected ROI. In this retrospective study, the models were developed and tested on the dataset acquired between April 2005 to April 2020. The additional independent dataset acquired at a different hospital between January 2016 to February 2019 was used for the external test of the classification model. Detection performance was assessed by mean average precision (mAP). Classification performance was assessed by the area under the receiver operating characteristic (AUROC), sensitivity, specificity, and Youden's index. 95% confidence intervals were calculated via non-parametric bootstrap to assess the statistical significance of model performances. Results: The ROI detection model achieved mAP of 0.819 at 0.75 intersection over union (IoU) thresholds in the internal test. In internal and external tests, the ADD classification model achieved AUROC values of 0.985 and 0.960, sensitivities of 0.950 and 0.926, and specificities of 0.919 and 0.892, respectively. Conclusions: The proposed explainable deep learning-based engine provides clinicians with the predictive result and its visualized rationale. The clinicians can make the final diagnosis by integrating primary diagnostic prediction obtained from the proposed engine with the patient's clinical examination findings. & COPY; 2023 Elsevier B.V. All rights reserved.
- DOI
- 10.1016/j.cmpb.2023.107465
- Appears in Collections:
- 의과대학 > 의학과 > Journal papers
- Files in This Item:
There are no files associated with this item.
- Export
- RIS (EndNote)
- XLS (Excel)
- XML