View : 441 Download: 0
On the Confidence of Stereo Matching in a Deep-Learning Era: A Quantitative Evaluation
- Title
- On the Confidence of Stereo Matching in a Deep-Learning Era: A Quantitative Evaluation
- Authors
- Poggi, Matteo; Kim, Seungryong; Tosi, Fabio; Kim, Sunok; Aleotti, Filippo; Min, Dongbo; Sohn, Kwanghoon; Mattoccia, Stefano
- Ewha Authors
- 민동보
- SCOPUS Author ID
- 민동보
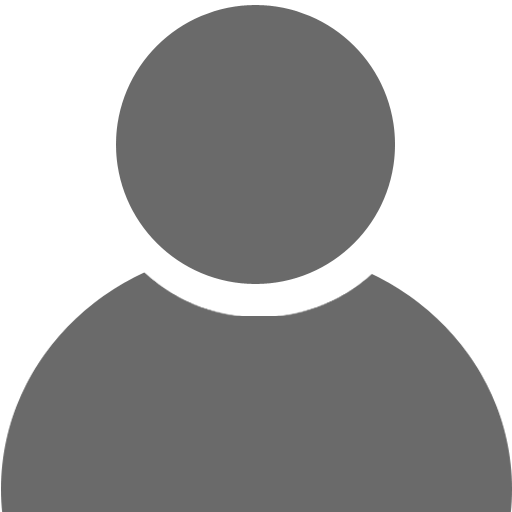
- Issue Date
- 2022
- Journal Title
- IEEE TRANSACTIONS ON PATTERN ANALYSIS AND MACHINE INTELLIGENCE
- ISSN
- 0162-8828
1939-3539
- Citation
- IEEE TRANSACTIONS ON PATTERN ANALYSIS AND MACHINE INTELLIGENCE vol. 44, no. 9, pp. 5293 - 5313
- Keywords
- Time measurement; Volume measurement; Estimation; Reliability; Q measurement; Pipelines; Machine learning algorithms; Stereo matching; confidence measures; machine learning; deep learning
- Publisher
- IEEE COMPUTER SOC
- Indexed
- SCIE; SCOPUS

- Document Type
- Article
- Abstract
- Stereo matching is one of the most popular techniques to estimate dense depth maps by finding the disparity between matching pixels on two, synchronized and rectified images. Alongside with the development of more accurate algorithms, the research community focused on finding good strategies to estimate the reliability, i.e., the confidence, of estimated disparity maps. This information proves to be a powerful cue to naively find wrong matches as well as to improve the overall effectiveness of a variety of stereo algorithms according to different strategies. In this paper, we review more than ten years of developments in the field of confidence estimation for stereo matching. We extensively discuss and evaluate existing confidence measures and their variants, from hand-crafted ones to the most recent, state-of-the-art learning based methods. We study the different behaviors of each measure when applied to a pool of different stereo algorithms and, for the first time in literature, when paired with a state-of-the-art deep stereo network. Our experiments, carried out on five different standard datasets, provide a comprehensive overview of the field, highlighting in particular both strengths and limitations of learning-based strategies.
- DOI
- 10.1109/TPAMI.2021.3069706
- Appears in Collections:
- 인공지능대학 > 컴퓨터공학과 > Journal papers
- Files in This Item:
There are no files associated with this item.
- Export
- RIS (EndNote)
- XLS (Excel)
- XML