View : 572 Download: 148
Predicting preterm birth through vaginal microbiota, cervical length, and WBC using a machine learning model
- Title
- Predicting preterm birth through vaginal microbiota, cervical length, and WBC using a machine learning model
- Authors
- Park, Sunwha; Moon, Jeongsup; Kang, Nayeon; Kim, Young-Han; You, Young-Ah; Kwon, Eunjin; Ansari, AbuZar; Hur, Young Min; Park, Taesung; Kim, Young Ju
- Ewha Authors
- 김영주; 유영아; Ansari Abuzar; 박선화
- SCOPUS Author ID
- 김영주
; 유영아
; Ansari Abuzar
; 박선화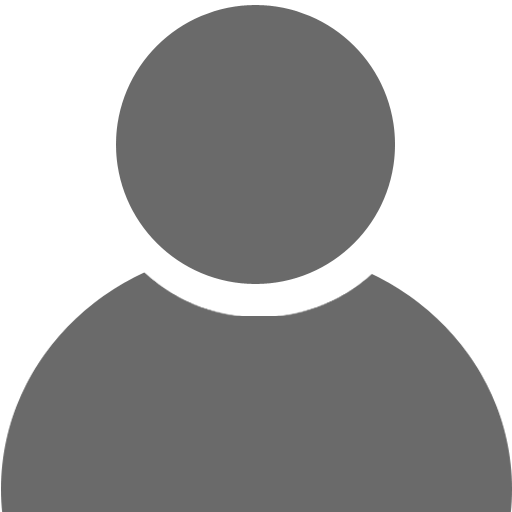
- Issue Date
- 2022
- Journal Title
- FRONTIERS IN MICROBIOLOGY
- ISSN
- 1664-302X
- Citation
- FRONTIERS IN MICROBIOLOGY vol. 13
- Keywords
- preterm birth; vaginal microbiome; pregnancy; 16s ribosomal RNA metagenome sequencing; cervicovaginal fluid; machine learning; microbial-marker
- Publisher
- FRONTIERS MEDIA SA
- Indexed
- SCIE; SCOPUS

- Document Type
- Article
- Abstract
- An association between the vaginal microbiome and preterm birth has been reported. However, in practice, it is difficult to predict premature birth using the microbiome because the vaginal microbial community varies highly among samples depending on the individual, and the prediction rate is very low. The purpose of this study was to select markers that improve predictive power through machine learning among various vaginal microbiota and develop a prediction algorithm with better predictive power that combines clinical information. As a multicenter case-control study with 150 Korean pregnant women with 54 preterm delivery group and 96 full-term delivery group, cervicovaginal fluid was collected from pregnant women during mid-pregnancy. Their demographic profiles (age, BMI, education level, and PTB history), white blood cell count, and cervical length were recorded, and the microbiome profiles of the cervicovaginal fluid were analyzed. The subjects were randomly divided into a training (n = 101) and a test set (n = 49) in a two-to-one ratio. When training ML models using selected markers, five-fold cross-validation was performed on the training set. A univariate analysis was performed to select markers using seven statistical tests, including the Wilcoxon rank-sum test. Using the selected markers, including Lactobacillus spp., Gardnerella vaginalis, Ureaplasma parvum, Atopobium vaginae, Prevotella timonensis, and Peptoniphilus grossensis, machine learning models (logistic regression, random forest, extreme gradient boosting, support vector machine, and GUIDE) were used to build prediction models. The test area under the curve of the logistic regression model was 0.72 when it was trained with the 17 selected markers. When analyzed by combining white blood cell count and cervical length with the seven vaginal microbiome markers, the random forest model showed the highest test area under the curve of 0.84. The GUIDE, the single tree model, provided a more reasonable biological interpretation, using the 10 selected markers (A. vaginae, G. vaginalis, Lactobacillus crispatus, Lactobacillus fornicalis, Lactobacillus gasseri, Lactobacillus iners, Lactobacillus jensenii, Peptoniphilus grossensis, P. timonensis, and U. parvum), and the covariates produced a tree with a test area under the curve of 0.77. It was confirmed that the association with preterm birth increased when P. timonensis and U. parvum increased (AUC = 0.77), which could also be explained by the fact that as the number of Peptoniphilus lacrimalis increased, the association with preterm birth was high (AUC = 0.77). Our study demonstrates that several candidate bacteria could be used as potential predictors for preterm birth, and that the predictive rate can be increased through a machine learning model employing a combination of cervical length and white blood cell count information.
- DOI
- 10.3389/fmicb.2022.912853
- Appears in Collections:
- 의과대학 > 의학과 > Journal papers
- Files in This Item:
-
fmicb-13-912853.pdf(1.36 MB)
Download
- Export
- RIS (EndNote)
- XLS (Excel)
- XML