View : 324 Download: 0
Forecasting of non-accidental, cardiovascular, and respiratory mortality with environmental exposures adopting machine learning approaches
- Title
- Forecasting of non-accidental, cardiovascular, and respiratory mortality with environmental exposures adopting machine learning approaches
- Authors
- Lee W.; Lim Y.-H.; Ha E.; Kim Y.; Lee W.K.
- Ewha Authors
- 하은희
- SCOPUS Author ID
- 하은희
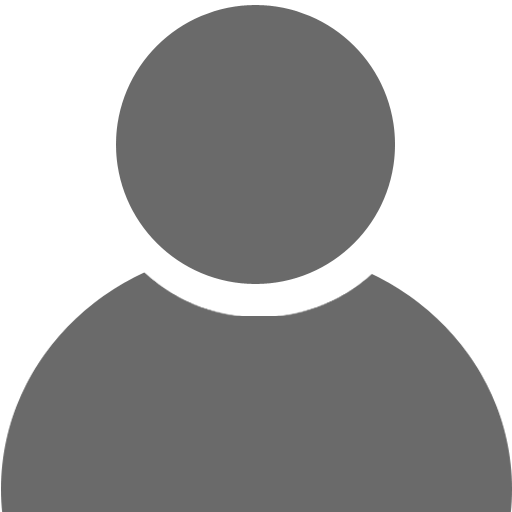
- Issue Date
- 2022
- Journal Title
- Environmental Science and Pollution Research
- ISSN
- 0944-1344
- Citation
- Environmental Science and Pollution Research vol. 29, no. 58, pp. 88318 - 88329
- Keywords
- Cardiovascular diseases; Deep learning; Environmental exposures; Machine learning; Respiratory tract diseases
- Publisher
- Springer Science and Business Media Deutschland GmbH
- Indexed
- SCIE; SCOPUS

- Document Type
- Article
- Abstract
- Environmental exposure constantly changes with time and various interactions that can affect health outcomes. Machine learning (ML) or deep learning (DL) algorithms have been used to solve complex problems, such as multiple exposures and their interactions. This study developed predictive models for cause-specific mortality using ML and DL algorithms with the daily or hourly measured meteorological and air pollution data. The ML algorithm improved the performance compared to the conventional methods, even though the optimal algorithm depended on the adverse health outcomes. The best algorithms were extreme gradient boosting, ridge, and elastic net, respectively, for non-accidental, cardiovascular, and respiratory mortality with daily measurement; they were superior to the generalized additive model reducing a mean absolute error by 4.7%, 4.9%, and 16.8%, respectively. With hourly measurements, the ML model tended to outperform the conventional models, even though hourly data, instead of daily data, did not enhance the performance in some models. The proposed model allows a better understanding and development of robust predictive models for health outcomes using multiple environmental exposures. © 2022, The Author(s), under exclusive licence to Springer-Verlag GmbH Germany, part of Springer Nature.
- DOI
- 10.1007/s11356-022-21768-9
- Appears in Collections:
- 의과대학 > 의학과 > Journal papers
- Files in This Item:
There are no files associated with this item.
- Export
- RIS (EndNote)
- XLS (Excel)
- XML