View : 375 Download: 0
Artificial intelligence predicts clinically relevant atrial high-rate episodes in patients with cardiac implantable electronic devices
- Title
- Artificial intelligence predicts clinically relevant atrial high-rate episodes in patients with cardiac implantable electronic devices
- Authors
- Kim M.; Kang Y.; You S.C.; Park H.-D.; Lee S.-S.; Kim T.-H.; Yu H.T.; Choi E.-K.; Park H.-S.; Park J.; Lee Y.S.; Kang K.-W.; Shim J.; Sung J.-H.; Oh I.-Y.; Park J.S.; Joung B.
- Ewha Authors
- 박준범
- SCOPUS Author ID
- 박준범
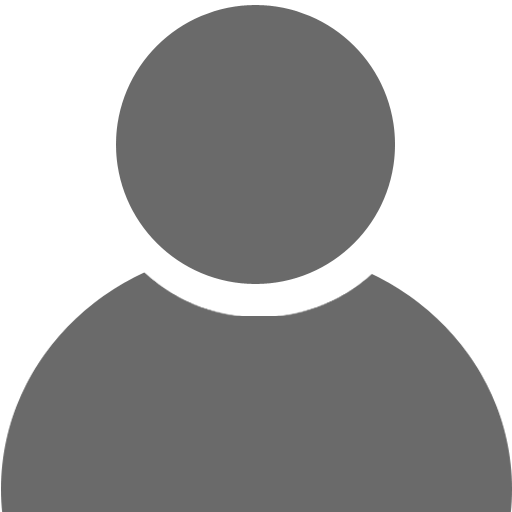
- Issue Date
- 2022
- Journal Title
- Scientific Reports
- ISSN
- 2045-2322
- Citation
- Scientific Reports vol. 12, no. 1
- Publisher
- Nature Research
- Indexed
- SCIE; SCOPUS

- Document Type
- Article
- Abstract
- To assess the utility of machine learning (ML) algorithms in predicting clinically relevant atrial high-rate episodes (AHREs), which can be recorded by a pacemaker. We aimed to develop ML-based models to predict clinically relevant AHREs based on the clinical parameters of patients with implanted pacemakers in comparison to logistic regression (LR). We included 721 patients without known atrial fibrillation or atrial flutter from a prospective multicenter (11 tertiary hospitals) registry comprising all geographical regions of Korea from September 2017 to July 2020. Predictive models of clinically relevant AHREs were developed using the random forest (RF) algorithm, support vector machine (SVM) algorithm, and extreme gradient boosting (XGB) algorithm. Model prediction training was conducted by seven hospitals, and model performance was evaluated using data from four hospitals. During a median follow-up of 18 months, clinically relevant AHREs were noted in 104 patients (14.4%). The three ML-based models improved the discrimination of the AHREs (area under the receiver operating characteristic curve: RF: 0.742, SVM: 0.675, and XGB: 0.745 vs. LR: 0.669). The XGB model had a greater resolution in the Brier score (RF: 0.008, SVM: 0.008, and XGB: 0.021 vs. LR: 0.013) than the other models. The use of the ML-based models in patient classification was associated with improved prediction of clinically relevant AHREs after pacemaker implantation. © 2022, The Author(s).
- DOI
- 10.1038/s41598-021-03914-4
- Appears in Collections:
- 의과대학 > 의학과 > Journal papers
- Files in This Item:
There are no files associated with this item.
- Export
- RIS (EndNote)
- XLS (Excel)
- XML