View : 518 Download: 0
Human Action Recognition: A Paradigm of Best Deep Learning Features Selection and Serial Based Extended Fusion
- Title
- Human Action Recognition: A Paradigm of Best Deep Learning Features Selection and Serial Based Extended Fusion
- Authors
- Khan, Seemab; Khan, Muhammad Attique; Alhaisoni, Majed; Tariq, Usman; Yong, Hwan-Seung; Armghan, Ammar; Alenezi, Fayadh
- Ewha Authors
- 용환승
- SCOPUS Author ID
- 용환승
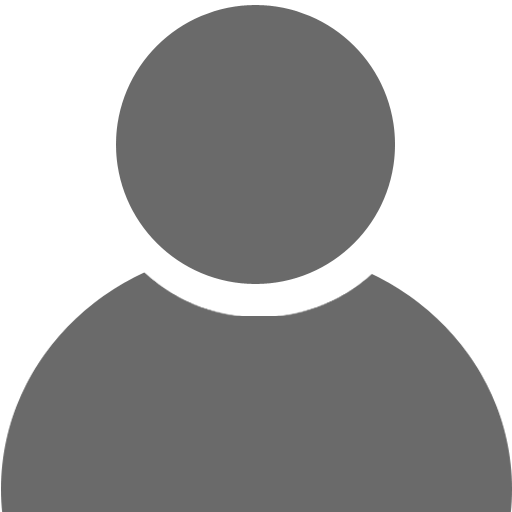
- Issue Date
- 2021
- Journal Title
- SENSORS
- ISSN
- 1424-8220
- Citation
- SENSORS vol. 21, no. 23
- Keywords
- human action recognition; deep learning; features fusion; features selection; recognition
- Publisher
- MDPI
- Indexed
- SCIE; SCOPUS

- Document Type
- Article
- Abstract
- Human action recognition (HAR) has gained significant attention recently as it can be adopted for a smart surveillance system in Multimedia. However, HAR is a challenging task because of the variety of human actions in daily life. Various solutions based on computer vision (CV) have been proposed in the literature which did not prove to be successful due to large video sequences which need to be processed in surveillance systems. The problem exacerbates in the presence of multi-view cameras. Recently, the development of deep learning (DL)-based systems has shown significant success for HAR even for multi-view camera systems. In this research work, a DL-based design is proposed for HAR. The proposed design consists of multiple steps including feature mapping, feature fusion and feature selection. For the initial feature mapping step, two pre-trained models are considered, such as DenseNet201 and InceptionV3. Later, the extracted deep features are fused using the Serial based Extended (SbE) approach. Later on, the best features are selected using Kurtosis-controlled Weighted KNN. The selected features are classified using several supervised learning algorithms. To show the efficacy of the proposed design, we used several datasets, such as KTH, IXMAS, WVU, and Hollywood. Experimental results showed that the proposed design achieved accuracies of 99.3%, 97.4%, 99.8%, and 99.9%, respectively, on these datasets. Furthermore, the feature selection step performed better in terms of computational time compared with the state-of-the-art.
- DOI
- 10.3390/s21237941
- Appears in Collections:
- 인공지능대학 > 컴퓨터공학과 > Journal papers
- Files in This Item:
There are no files associated with this item.
- Export
- RIS (EndNote)
- XLS (Excel)
- XML