View : 699 Download: 0
A feature selection method for classifying highly similar text documents
- Title
- A feature selection method for classifying highly similar text documents
- Authors
- Kim J.; Min D.
- Ewha Authors
- 민대기
- SCOPUS Author ID
- 민대기
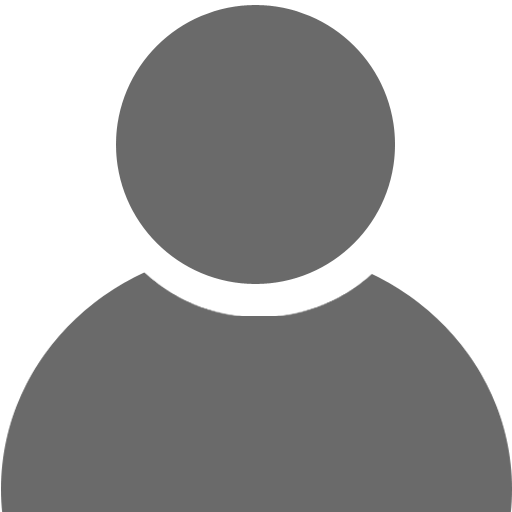
- Issue Date
- 2021
- Journal Title
- Industrial Engineering and Management Systems
- ISSN
- 1598-7248
- Citation
- Industrial Engineering and Management Systems vol. 20, no. 2, pp. 148 - 162
- Keywords
- Feature selection; Hierarchical classification; Multi-classification; Overlapping feature
- Publisher
- Korean Institute of Industrial Engineers
- Indexed
- SCOPUS; KCI

- Document Type
- Article
- Abstract
- In the era of big data, the importance of data classification is increasing. However, when it comes to classifying text documents, several obstacles degrade classification performance. These include multi-class documents, high levels of similarity between classes, class size imbalance, high dimensional representation space, and a low frequency of unique and discriminative features. To overcome these obstacles and improve classification performance, this paper proposes a novel feature selection method that effectively utilizes both unique and overlapping features. In general, feature selection methods have ignored unique features that occur only one class because of low frequency while it provides better discriminative-power. On the contrary, overlapping features, which are found in several classes with high frequency, have been also less preferred because of low discriminative-power. The proposed feature selection method attempts to use these two types of features as complementary with aims to improve overall classification performance for highly similar text documents. Extensive numerical analysis have been conducted for three benchmarking datasets with a support vector machine (SVM) classifier. The proposed method showed that not only the class with high similarity but also the general classification performance is superior to the conventional feature selection methods, such as the global feature set, local feature set, discriminative feature set, and information gain. © 2021 KIIE.
- DOI
- 10.7232/iems.2021.20.2.148
- Appears in Collections:
- 경영대학 > 경영학전공 > Journal papers
- Files in This Item:
There are no files associated with this item.
- Export
- RIS (EndNote)
- XLS (Excel)
- XML