View : 451 Download: 0
Clustered Manifold Approximation and Projection for Semisupervised Fault Diagnosis and Process Monitoring
- Title
- Clustered Manifold Approximation and Projection for Semisupervised Fault Diagnosis and Process Monitoring
- Authors
- Park, Damdae; Na, Jonggeol; Lee, Jong Min
- Ewha Authors
- 나종걸
- SCOPUS Author ID
- 나종걸
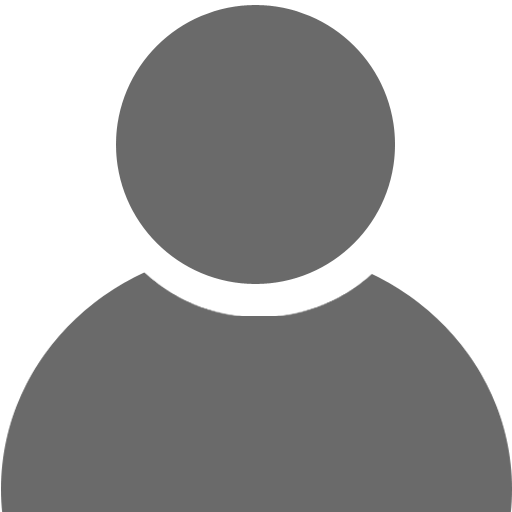
- Issue Date
- 2021
- Journal Title
- INDUSTRIAL & ENGINEERING CHEMISTRY RESEARCH
- ISSN
- 0888-5885
- Citation
- INDUSTRIAL & ENGINEERING CHEMISTRY RESEARCH vol. 60, no. 26, pp. 9521 - 9531
- Publisher
- AMER CHEMICAL SOC
- Indexed
- SCIE; SCOPUS

- Document Type
- Article
- Abstract
- With increasing demands on product quality and safety requirements, modern industrial processes are highly instrumented and the data collected are being utilized to monitor and diagnose processes. In many cases, the process records include labels that indicate process operating conditions or prior knowledge of the sample points, which can be used to improve diagnostic performance. For this reason, semisupervised methods that can utilize both labeled and unlabeled data are recently gaining interest. In this article, we propose a novel manifold learning-based semisupervised process monitoring method, named Clustered Manifold Approximation and Projection (CMAP). In CMAP, a data manifold is approximated ahead of projection, where the distance on the manifold is defined by the pairwise interaction between the data points induced by metric and nonmetric attributes. This allows simultaneous utilization of limited labeled data and abundant unlabeled data, as well as enables tracking and controlling their effect on the projection. By postulating a well-behaved manifold that preserves discriminant and temporal characteristics of the process, CMAP shows superior performance in the process monitoring and fault diagnosis tasks. The effectiveness of the proposed method is assessed on a dataset obtained from the Tennessee Eastman process and compared with five competing methods.
- DOI
- 10.1021/acs.iecr.1c01271
- Appears in Collections:
- 공과대학 > 화공신소재공학과 > Journal papers
- Files in This Item:
There are no files associated with this item.
- Export
- RIS (EndNote)
- XLS (Excel)
- XML