View : 480 Download: 0
Automatic segmentation of multiple cardiovascular structures from cardiac computed tomography angiography images using deep learning
- Title
- Automatic segmentation of multiple cardiovascular structures from cardiac computed tomography angiography images using deep learning
- Authors
- Baskaran, Lohendran; Al'Aref, Subhi J.; Maliakal, Gabriel; Lee, Benjamin C.; Xu, Zhuoran; Choi, Jeong W.; Lee, Sang-Eun; Sung, Ji Min; Lin, Fay Y.; Dunham, Simon; Mosadegh, Bobak; Kim, Yong-Jin; Gottlieb, Ilan; Lee, Byoung Kwon; Chun, Eun Ju; Cademartiri, Filippo; Maffei, Erica; Marques, Hugo; Shin, Sanghoon; Choi, Jung Hyun; Chinnaiyan, Kavitha; Hadamitzky, Martin; Conte, Edoardo; Andreini, Daniele; Pontone, Gianluca; Budoff, Matthew J.; Leipsic, Jonathon A.; Raff, Gilbert L.; Virmani, Renu; Samady, Habib; Stone, Peter H.; Berman, Daniel S.; Narula, Jagat; Bax, Jeroen J.; Chang, Hyuk-Jae; Min, James K.; Shaw, Leslee J.
- Ewha Authors
- 신상훈; 이상은
- SCOPUS Author ID
- 신상훈
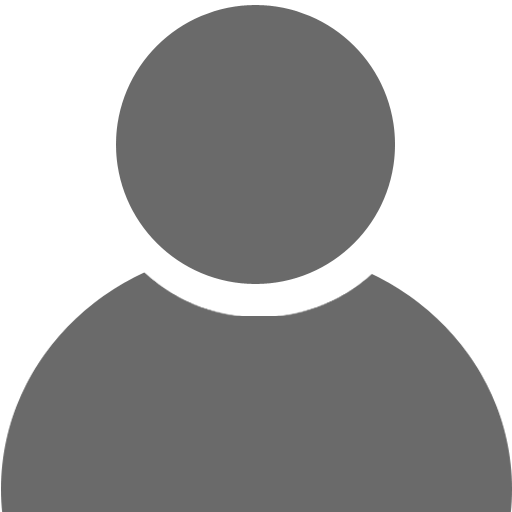
; 이상은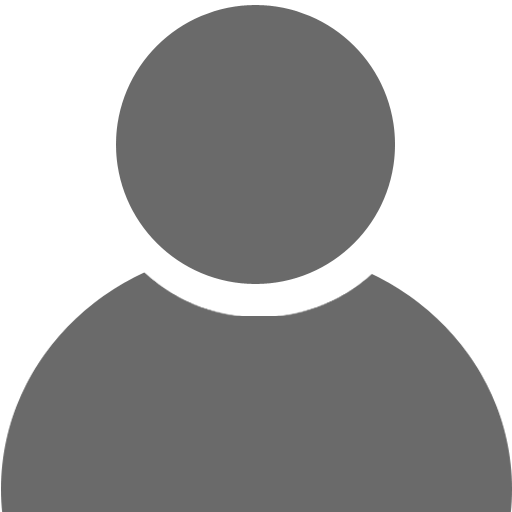
- Issue Date
- 2020
- Journal Title
- PLOS ONE
- ISSN
- 1932-6203
- Citation
- PLOS ONE vol. 15, no. 5
- Publisher
- PUBLIC LIBRARY SCIENCE
- Indexed
- SCIE; SCOPUS

- Document Type
- Article
- Abstract
- Background Segmentation of cardiovascular images is resource-intensive. We design an automated deep learning method for the segmentation of multiple structures from Coronary Computed Tomography Angiography (CCTA) images. Methods Images from a multicenter registry of patients that underwent clinically-indicated CCTA were used. The proximal ascending and descending aorta (PAA, DA), superior and inferior vena cavae (SVC, IVC), pulmonary artery (PA), coronary sinus (CS), right ventricular wall (RVW) and left atrial wall (LAW) were annotated as ground truth. The U-net-derived deep learning model was trained, validated and tested in a 70:20:10 split. Results The dataset comprised 206 patients, with 5.130 billion pixels. Mean age was 59.9 +/- 9.4 yrs., and was 42.7% female. An overall median Dice score of 0.820 (0.782, 0.843) was achieved. Median Dice scores for PAA, DA, SVC, IVC, PA, CS, RVW and LAW were 0.969 (0.979, 0.988), 0.953 (0.955, 0.983), 0.937 (0.934, 0.965), 0.903 (0.897, 0.948), 0.775 (0.724, 0.925), 0.720 (0.642, 0.809), 0.685 (0.631, 0.761) and 0.625 (0.596, 0.749) respectively. Apart from the CS, there were no significant differences in performance between sexes or age groups. Conclusions An automated deep learning model demonstrated segmentation of multiple cardiovascular structures from CCTA images with reasonable overall accuracy when evaluated on a pixel level.
- DOI
- 10.1371/journal.pone.0232573
- Appears in Collections:
- 의료원 > 의료원 > Journal papers
- Files in This Item:
There are no files associated with this item.
- Export
- RIS (EndNote)
- XLS (Excel)
- XML