View : 500 Download: 0
Deep Convolutional Neural Network Based Interictal-Preictal Electroencephalography Prediction: Application to Focal Cortical Dysplasia Type-II
- Title
- Deep Convolutional Neural Network Based Interictal-Preictal Electroencephalography Prediction: Application to Focal Cortical Dysplasia Type-II
- Authors
- Chung Y.G.; Jeon Y.; Choi S.A.; Cho A.; Kim H.; Hwang H.; Kim K.J.
- Ewha Authors
- 최선아
- SCOPUS Author ID
- 최선아
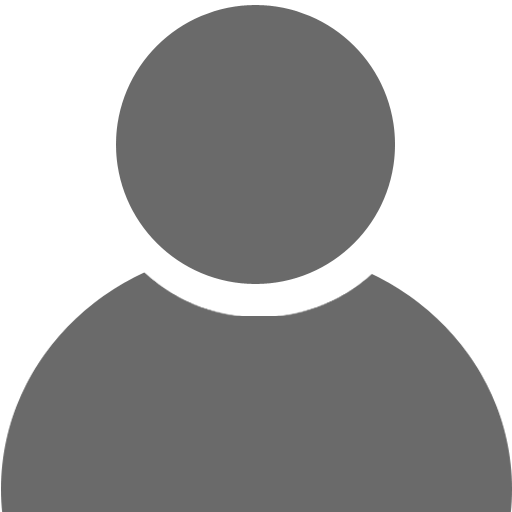
- Issue Date
- 2020
- Journal Title
- Frontiers in Neurology
- ISSN
- 1664-2295
- Citation
- Frontiers in Neurology vol. 11
- Keywords
- convolutional neural networks; deep learning; epilepsy surgery; focal cortical dysplasia; seizure prediction
- Publisher
- Frontiers Media S.A.
- Indexed
- SCIE; SCOPUS

- Document Type
- Article
- Abstract
- We aimed to differentiate between the interictal and preictal states in epilepsy patients with focal cortical dysplasia (FCD) type-II using deep learning-based classifiers based on intracranial electroencephalography (EEG). We also investigated the practical conditions for high interictal-preictal discriminability in terms of spatiotemporal EEG characteristics and data size efficiency. Intracranial EEG recordings of nine epilepsy patients with FCD type-II (four female, five male; mean age: 10.7 years) were analyzed. Seizure onset and channel ranking were annotated by two epileptologists. We performed three consecutive interictal-preictal classification steps by varying the preictal length, number of electrodes, and sampling frequency with convolutional neural networks (CNN) using 30 s time-frequency data matrices. Classification performances were evaluated based on accuracy, F1 score, precision, and recall with respect to the above-mentioned three parameters. We found that (1) a 5 min preictal length provided the best classification performance, showing a remarkable enhancement of >13% on average compared to that with the 120 min preictal length; (2) four electrodes provided considerably high classification performance with a decrease of only approximately 1% on average compared to that with all channels; and (3) there was minimal performance change when quadrupling the sampling frequency from 128 Hz. Patient-specific performance variations were noticeable with respect to the preictal length, and three patients showed above-average performance enhancements of >28%. However, performance enhancements were low with respect to both the number of electrodes and sampling frequencies, and some patients showed at most 1–2% performance change. CNN-based classifiers from intracranial EEG recordings using a small number of electrodes and efficient sampling frequency are feasible for predicting the interictal-preictal state transition preceding seizures in epilepsy patients with FCD type-II. Preictal lengths affect the predictability in a patient-specific manner; therefore, pre-examinations for optimal preictal length will be helpful in seizure prediction. © Copyright © 2020 Chung, Jeon, Choi, Cho, Kim, Hwang and Kim.
- DOI
- 10.3389/fneur.2020.594679
- Appears in Collections:
- 의료원 > 의료원 > Journal papers
- Files in This Item:
There are no files associated with this item.
- Export
- RIS (EndNote)
- XLS (Excel)
- XML