View : 588 Download: 0
A Performance Comparison between Automated Deep Learning and Dental Professionals in Classification of Dental Implant Systems from Dental Imaging: A Multi-Center Study
- Title
- A Performance Comparison between Automated Deep Learning and Dental Professionals in Classification of Dental Implant Systems from Dental Imaging: A Multi-Center Study
- Authors
- Lee, Jae-Hong; Kim, Young-Taek; Lee, Jong-Bin; Jeong, Seong-Nyum
- Ewha Authors
- 이종빈
- SCOPUS Author ID
- 이종빈
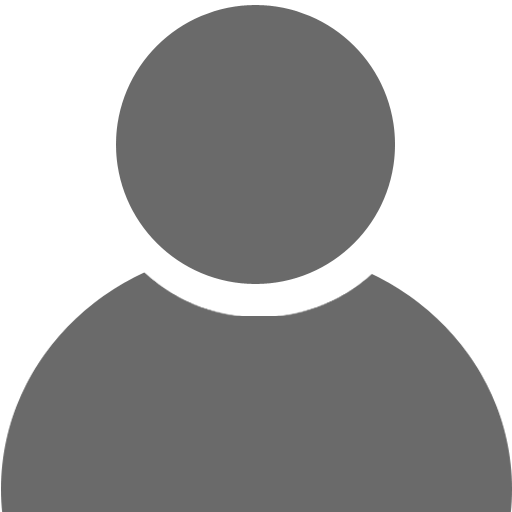
- Issue Date
- 2020
- Journal Title
- DIAGNOSTICS
- ISSN
- 2075-4418
- Citation
- DIAGNOSTICS vol. 10, no. 11
- Keywords
- artificial intelligence; dental implants; deep learning; supervised machine learning
- Publisher
- MDPI
- Indexed
- SCIE; SCOPUS

- Document Type
- Article
- Abstract
- In this study, the efficacy of the automated deep convolutional neural network (DCNN) was evaluated for the classification of dental implant systems (DISs) and the accuracy of the performance was compared against that of dental professionals using dental radiographic images collected from three dental hospitals. A total of 11,980 panoramic and periapical radiographic images with six different types of DISs were divided into training (n = 9584) and testing (n = 2396) datasets. To compare the accuracy of the trained automated DCNN with dental professionals (including six board-certified periodontists, eight periodontology residents, and 11 residents not specialized in periodontology), 180 images were randomly selected from the test dataset. The accuracy of the automated DCNN based on the AUC, Youden index, sensitivity, and specificity, were 0.954, 0.808, 0.955, and 0.853, respectively. The automated DCNN outperformed most of the participating dental professionals, including board-certified periodontists, periodontal residents, and residents not specialized in periodontology. The automated DCNN was highly effective in classifying similar shapes of different types of DISs based on dental radiographic images. Further studies are necessary to determine the efficacy and feasibility of applying an automated DCNN in clinical practice.
- DOI
- 10.3390/diagnostics10110910
- Appears in Collections:
- 의료원 > 의료원 > Journal papers
- Files in This Item:
There are no files associated with this item.
- Export
- RIS (EndNote)
- XLS (Excel)
- XML