View : 838 Download: 0
Automated Skeletal Classification with Lateral Cephalometry Based on Artificial Intelligence
- Title
- Automated Skeletal Classification with Lateral Cephalometry Based on Artificial Intelligence
- Authors
- Yu, H. J.; Cho, S. R.; Kim, M. J.; Kim, W. H.; Kim, J. W.; Choi, J.
- Ewha Authors
- 김민지; 김진우
- SCOPUS Author ID
- 김민지
; 김진우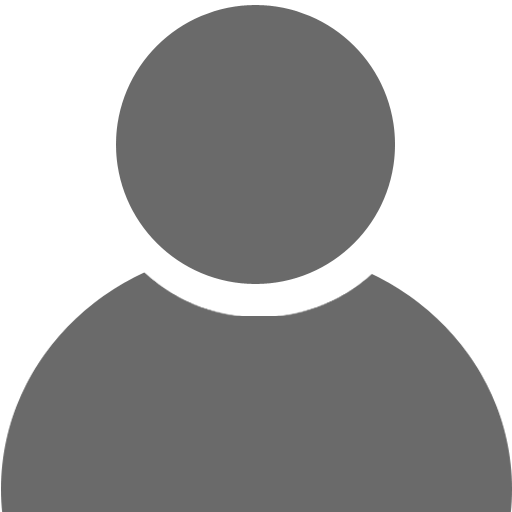
- Issue Date
- 2020
- Journal Title
- JOURNAL OF DENTAL RESEARCH
- ISSN
- 0022-0345
1544-0591
- Citation
- JOURNAL OF DENTAL RESEARCH vol. 99, no. 3, pp. 249 - 256
- Keywords
- deep learning; orthodontics; diagnosis; orthognathic surgery; diagnostic imaging; neural networks
- Publisher
- SAGE PUBLICATIONS INC
- Indexed
- SCIE; SCOPUS

- Document Type
- Article
- Abstract
- Lateral cephalometry has been widely used for skeletal classification in orthodontic diagnosis and treatment planning. However, this conventional system, requiring manual tracing of individual landmarks, contains possible errors of inter- and intravariability and is highly time-consuming. This study aims to provide an accurate and robust skeletal diagnostic system by incorporating a convolutional neural network (CNN) into a 1-step, end-to-end diagnostic system with lateral cephalograms. A multimodal CNN model was constructed on the basis of 5,890 lateral cephalograms and demographic data as an input. The model was optimized with transfer learning and data augmentation techniques. Diagnostic performance was evaluated with statistical analysis. The proposed system exhibited >90% sensitivity, specificity, and accuracy for vertical and sagittal skeletal diagnosis. Clinical performance of the vertical classification showed the highest accuracy at 96.40 (95% CI, 93.06 to 98.39; model III). The receiver operating characteristic curve and the area under the curve both demonstrated the excellent performance of the system, with a mean area under the curve >95%. The heat maps of cephalograms were also provided for deeper understanding of the quality of the learned model by visually representing the region of the cephalogram that is most informative in distinguishing skeletal classes. In addition, we present broad applicability of this system through subtasks. The proposed CNN-incorporated system showed potential for skeletal orthodontic diagnosis without the need for intermediary steps requiring complicated diagnostic procedures.
- DOI
- 10.1177/0022034520901715
- Appears in Collections:
- 의과대학 > 의학과 > Journal papers
- Files in This Item:
There are no files associated with this item.
- Export
- RIS (EndNote)
- XLS (Excel)
- XML